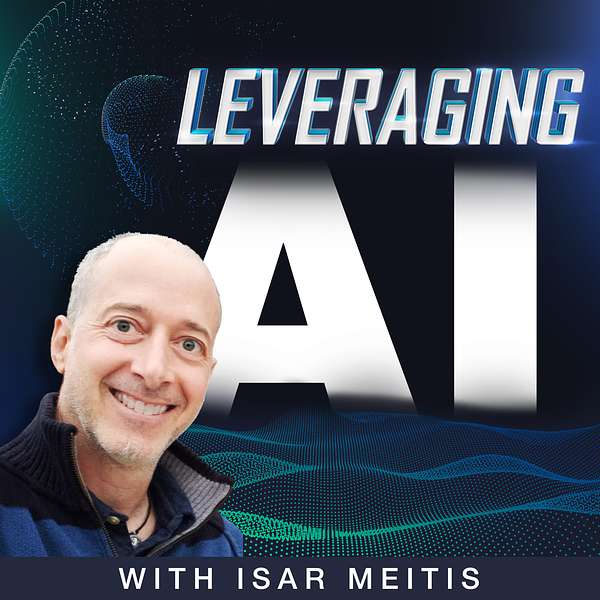
Leveraging AI
Dive into the world of artificial intelligence with 'Leveraging AI,' a podcast tailored for forward-thinking business professionals. Each episode brings insightful discussions on how AI can ethically transform business practices, offering practical solutions to day-to-day business challenges.
Join our host Isar Meitis (4 time CEO), and expert guests as they turn AI's complexities into actionable insights, and explore its ethical implications in the business world. Whether you are an AI novice or a seasoned professional, 'Leveraging AI' equips you with the knowledge and tools to harness AI's power responsibly and effectively. Tune in weekly for inspiring conversations and real-world applications. Subscribe now and unlock the potential of AI in your business.
Leveraging AI
179 | Beyond the Buzz: How to create AI Agents that provide Real Business value with Tom Winter
AI Agent hype is everywhere—but what does it actually take to build an AI agent that delivers results? Not just one that chats back in clever text, but one that does real, meaningful work inside your business processes.
In this live, tactical session, we’re walking you through the step-by-step method to combine workflows and agents into real-world automations. From defining where agents fit, to layering in “critic stages” that improve quality, to setting up humans-in-the-loop when needed—this is your no-fluff guide to operational AI.
Tom Winter, Co-Founder of SEOwind and a true practitioner when it comes to building intelligent systems that scale. He doesn't just talk about AI—he codes it, tests it 150 times, and knows what breaks (and how to fix it). With years of experience applying automation across SaaS, SEO, and content creation, Tom will reveal the frameworks, prompts, and hidden tricks that actually work.
If you're tired of the hype and ready to build smarter, this session is for you.
🚀 Learn more about the AI Business Transformation Course (link in show notes) — now $150 off with promo code HAPPYBIRTHDAY for a limited time. Perfect for business leaders looking to implement AI step-by-step, not just talk about it.
About Leveraging AI
- The Ultimate AI Course for Business People: https://multiplai.ai/ai-course/
- YouTube Full Episodes: https://www.youtube.com/@Multiplai_AI/
- Connect with Isar Meitis: https://www.linkedin.com/in/isarmeitis/
- Join our Live Sessions, AI Hangouts and newsletter: https://services.multiplai.ai/events
If you’ve enjoyed or benefited from some of the insights of this episode, leave us a five-star review on your favorite podcast platform, and let us know what you learned, found helpful, or liked most about this show!
Hello and welcome to a live episode of the Leveraging AI podcast, a podcast that shares practical, ethical ways to improve efficiency, grow your business and advance your career. This is Isar Maitish your host, and we have a really great episode for you today. First of all, It's my birthday and I don't think I've ever done a live episode of anything in my birthday. You know what I mean? Going live for like five years. So this is exciting by itself. second is we have a topic that is maybe the biggest buzz in the world right now, which is AI agents. So that's a big deal to me. as well. And so what are AI agents? And everybody's really confused because everybody now calls everything AI agents. Literally anybody calls any automation or anything in AI agent because it's a buzzword and everybody wants agents. And that's not really true. Not everything is an agent. So we're going to talk a little bit about what are agents and how they're different than just AI or just AI with automation and we're gonna show you specific examples on how you need to think and what are the processes and what are the steps you need to take I'm sure you do if you listening to this podcast that at least cross your mind or that something you heard and you want to understand what it is. Now, I guess today winter was the wrap up. He is the perfect person to give us that, to walk us through this topic for several different reasons, but his background, he has been on both the development side and the business running side for many years. So for many years, he was helping large organization skill up their development team to do things faster, better, and more efficient, which now he's doing with agents, but in the past few years, he's been running his own business. So he also understands what matters from a business standpoint. Value efficiency, cost reduction, and so on and so forth, which makes it really the perfect mix of understanding the technical side of things as well as the business side of things. So I'm personally very, very excited to hear what Tom has to say. I've actually seen some of the stuff that he's done in previous meetings we had. I've been on his podcast, so we went back and forth several times, so I'm very, very excited. Tom, thank you so much for joining us. Welcome to Leveraging AI. Thank
Tom Winter:you, sir, for such a warm welcome, and of course, happy birthday, all the best, and thank you for spending this time, like, on your birthday with me, which is really, really amazing. I've been watching you for a long time and really love what you're doing.
Isar Meitis:Thank you, thank you so much. This is, this is great. I, I really admire what you do as well. I think the, I didn't say this just to hype the audience. I really think having both these skills, the business skills and the technical skills, has always been important. But I think now it's, it's nothing short of a magic wand that allows you to do things that were literally impossible two years ago. and now you can do at scale. So I'm very, very excited about that. I want to thank everybody in the audience. That is our joining us live both on LinkedIn and on Zoom. So if you are here, please introduce yourself, say where you're from, say what you know about agents, what you want to know about agents. And at any point during this conversation, if you have any questions, please write them in the chat and we will answer them. to answer it and I will try to ask Tom in the right time to provide you answers. In addition, one more thing that I want to say, it is my birthday so I thought of how can I treat everybody as a birthday present for myself? So as you No, if you're listening to this podcast, the most important thing, the most impactful way you can promote your career and your capabilities and your company or team and so on is through better, more construct AI education. Now, the podcast is fantastic. We have people like Tom and we share really great value, but at the end of the day, to really implement this in a business environment, you need more, more people. than just listening to podcasts or following people on Twitter and tech talk or YouTube, wherever you follow different people, you need a very structured approach. And that's why we have the AI business transformation course that we have been running for two years now. So the first course started in April of 2023, we've been teaching this course at least once a month. So hundreds, maybe thousands of business people has went through this course right now. It's getting raving reviews and it's really getting people to drive. immediate results in their businesses. And we're opening our spring session of our course in on May 12th. And so why is that has to do with my birthday where I decided to make it fun and create the deepest discount we ever give, which is$150 on the course. So if you sign up, by the end of next week, after this goes live and becomes a podcast as well, by the end of next week, if you sign up and you use the link in the description, promo code happy birthday all caps one word you will get 150 dollars off the course now i know people listen from all over the world and people like oh my god this is in the u.s and you're in the east coast and i'm in wherever so the time zones don't work we had people in the course from new zealand australia india middle east Emirates, many places in Europe, obviously all across us and Hawaii. So more or less any time zone of the planet, we had people join the course and multiple people join the course and everybody were very happy about doing this. So don't think twice. Come join us. But now for the topic of today, I'm really excited Tom for what you have to share with us and the stage is yours. Walk us through your magic on how to think about the Agent implementation in a business perspective,
Tom Winter:Okay. So as Isar said, like I'm half a developer, half a marketer, and, I implement AI, like, This is my on daily basis, in our own tool. Right now I'm focused on a tool called SEO wind, which is. And AI writing tool, where we focus on the research part. So our main goal is to do the research to provide to AI context that it needs. And today I will be talking about beyond the bus, how to create agents that provide real business value. So to tell you about the agenda. Sampai jumpa! I will go through a automation essential. So tell you a little bit about high level stuff. Like what are Automations show you tell you about what are a workflows? What are agents like how they are different and then at the end I will go through exactly my process Like how I use an a workflow with a agents like in a hybrid approach to guide you what you can implement. This is an example that I want to walk you through And show you exactly how am I doing that. if Isar will have any questions in between, like definitely he can ask if you will have any questions, just pop it up, like add it to the chat. And definitely Isar will help you out with asking me these questions. So I'm happy to share all the knowledge. So AI automation essential. So the first thing that. I want to share before we go into AI automations. This is my opinion. This is my private opinion. If you don't implement AI now, you have to fire 100 percent of your staff in a year or two. Why am I saying that? Because I'm talking to a lot of people and whenever I'm telling them like, just implement some AI information, AI, things into your workflows, they're saying like, Oh, but we will have to fire 50 percent of our stuff if we do it. but think about it from a different perspective. If you don't help out your company to become better, to get a better R why from what you're doing to compete better on the market, your competition will do that. So this is the problem that I see definitely, that may have Impact your company in a year or two. So the first thing To which we have to look at, is the success depends on clear objectives. So whatever you want to automate, you need to have really clear objectives, like name them, write them down. If you don't have an objective, that means you don't know what you want to automate. and this is why it's so important to actually do it. Define the tasks you want to automate. Actually try doing these tasks manually. I call it HAS, so human as a service, So when we're talking about software as a service, we talk about automating things as a product, but I feel before you start automating, actually try to do it as a human. You can also go to Vanilla LLM, like ChatGPT, Cloud, whatever, but try to go through these steps. If you want to automate something that you don't know the objectives. Come on, what are the objectives? You don't know how the process works. What are you expecting from AI to do? So basically, if you expect AI to figure it all out, I'll say it in a harsh way, you're or I'm not needed basically for the process, but if AI will take over over everything, there. So I think really it's important to go through this whole process and understand what are the pitfalls of the, of the process. What are the edge cases? Do it slowly as a human. If you do it, then you can actually do more. Then there is a question between two, like, what are the differences between GPT's AI workflows and AI agents? Actually, I would add a fourth one here, which is vanilla LLM. So basically chat GPT Claude, so if we start with vanilla LLM, which would be left from GPTs, this is basically the chat. So you you're chatting with directly with LLMs and you're getting an answer based on whatever AI wants to answer you. Then you have GPTs. GPTs, I call it this way, probably there are more names for that. To this was the name that OpenAI gave in the beginning, basically they're kind of pre-trained, although I don't like to use the word trained. it's customized LLMs provided with specific knowledge that it helps to resolve a specific task. So you can go, for example, to OpenAI, there is a GPT store, there's plenty of GPTs there that are customized for a specific task. For example, it can be a task to Rewrite your LinkedIn posts maybe. proofread something. I've just used one for creating images. Anything that is like very defined for what the task should do. They're really amazing because they can bring results because they have like specific instructions inside. maybe there is an additional knowledge base inside that can help you out to resolve this specific task. But this is like kind of. selamat menikmati! Something that can resolve specific one task, just to help,
Isar Meitis:just to help people understand what this means, basically custom GPTs are a regular conversation that you want to do in a repetitive way. So any task that you want to do with Chachapiti again and again and again, instead of typing the prompt again and again and again, and giving it the. So the input could be, going back to Tom's idea, great ways to write a LinkedIn post. Great way to write hooks. What would be a good graphics to a LinkedIn? But like stuff like that, that is information that you would feed it every single time. Or if you're doing a business analysis, what's the template that we want to use for our analysis? What are the inputs that we're going to give it? Like all these things that you're going to just do. multiple times repetitively, you can build a custom GPT, that will do it for you. I'll just say one more thing. all the other tools have the same stuff, so you can build, it's called gems on Gemini. It's called spaces on perplexity. It's called like every one of these tools, Claude calls it, projects. So you can, you can build these mini automations and give them background information and instructions on all the different tools. It's not a specifically a, a, chat GPT thing. I still think Chachapiti has the best implementation right now and if you want there's an episode from two or three weeks ago that talks specifically about that that you can dive into my
Tom Winter:favorite name is at you.com they call it agents yes just to make it a little bit as we said in the beginning everybody calls everything agents now yeah exactly then you have ai workflows ai workflows are basically a process that you go through one by one to resolve a specific process but they are linear And, they happen basically one after the other to resolve a problem. which is also something that is really good, for solving more complex problems. Like, so they can be actually a combination of many GPTs together. they can be a combination of some executions, in between. And, but it's basically linear. So, like, One happens after the other and then there is AI agents. My definition of AI agents is a tool that it that resolves open ended questions without predefined. solution path. So basically it has a set of tools that it can use, and you ask it, a question and you want to resolve a specific problem. Then an AI agent creates the path to resolve the problem and then executes based on this path. the cool thing that I heard from ISAR, a while back in the future, is that Chyba chcemy zobaczyć agenty AI, któróje stworzymy agenty AI, więc nie będziemy musieli predefiniować agenty AI, ale, może będzie agenty AI, który stworzymy agenty AI dla nas, aby rozwiązać konkretne problemy. I kudos, myślę, że to jest przyszłość, zdecydowanie, którą zobaczymy. but understanding the process, like implementing AI is not magic. It's just basically structured and well-planned approach. So like if you have courses that you can join, like Isar shares it, definitely they will help you out to structure this approach, how to go, how to implement AI, and what kind of things do you need to implement to do it. So stage one is definitely this defining and discovering the process, then planning and designing. Thank you. Building and testing and launching and optimizing. And really, remember, it's not something that you just launch and forget you will have to optimize in the future and you will have to iterate on that. I want to pause you just for
Isar Meitis:just for one second in each and every one of the stages, so defining and discovering planning and designing, building and testing, and then launching and optimizing. You can use. All the other variants that Tom mentioned before, like just a regular chat or a custom GPT or whatever to help you in those processes. Like one of the things going back, you mentioned my courses. One of the things I teach in my courses is how to map business processes with AI. Something that used to take weeks and nobody did it because of that. So each and every person that listens to this podcast has in his company, some critical processes, not like a minor thing, critical processes that only Joe or Jill or Sam knows how to do. Thank you. And if God forbid they hit by a bus, then you'll have a serious problem because nobody knows there's no backup and we don't map these processes and document them because it's a lot of work. But AI can help you literally in each and every one of the steps that Tom mentioned. So yes, it's not the solution, but it can help you get to the solution significantly faster. And one more comment about the optimizing. optimizing is a very, very big deal. Because a, you will learn things after you make the first deployment and B, the AI tools themselves keep on getting better and better and have access to more and more tools. So everything that we do, we keep on changing like internally in our company, everything that we do, we keep on changing on regular basis, testing new models, testing new procedures, taking new connections. Oh my God, now it can do this. It couldn't have done this before. So this, this optimization process is never stopping. It's an evergreen process of optimization. I
Tom Winter:also have other ideas, because to be honest, is reasoning something new? Yes, it's implemented right now into many AI models, but was it possible a year ago? Yes, it was possible, you just had to divide the task into reasoning part and then solving part. Like it was doable, it was just not automated. So like, there's things changing that you can implement in an easier way once they're implemented in the models. And one crucial thing that I really think is important is something that I call cyber method. So basically, AI working with a human together, not against each other. We can help each other. We have superpowers. So humans have superpowers. AI has superpowers. Like our superpowers are, is our knowledge, our experience. We talk to the customers. we talk to the product team. We talk to sales marketing. Whoever we have in the company. so we have a lot of experience that we can add to AI. AI often doesn't know it. So in the whole process, you can actually have a human, involvement. And I think it's really, really useful. We used a lot when a writing. articles, using SEO wind, but definitely in every process, you can actually involve a human with their knowledge to be better in the whole process. Okay, so let's start with a workflows. So a workflows for me, like how I defined it is an AI powered assembly line. tak łatwo, więc krok po kroku dodając rzeczy do pracy. Dobre rzecz, kiedy chodzi o pracy, one pracują bardzo dobrze dla procesu biznesu zgodnie zdefiniowany, więc jeśli ty, tak jak powiedział Isar, jeśli masz to mapowane, pracują bardzo dobrze, ponieważ one idą point by point exactly going through the instructions so point one point two point three so they're i would say even better than humans because we as humans we like to optimize so if we have process one two three we like to skip process like the step number two because we think it's not necessary a workflow will actually follow it so this is really really good it simulates human-like process. So it's not that you will just go to AI and tell it like, do something, you will pour in like 300 pages of text and say like, I don't know, like let's resolve it. it's very similar to how you do it as a human. So when you're designing any workflow, you can think about it, how I would do it as a human, if I didn't have AI. To be honest, in my opinion, most important one, that repeat repeatable quality of the output. And whenever you need a repeatable quality of the output, that is, I'm not saying not creative, but like, it will bring you the similar results every single time, which is extremely hard when it comes to AI, because we love it for creativity, I would use AI workflows, this is exactly the thing that I would use it for. So when it comes to GPT's AI workflows versus agents, they're all really good, but for different types of tasks. So here, AI workflows really shine when it comes to repeatability of the process and the quality. So if you have, for example, a report for a customer and you have certain fields that you have to fill out, You wouldn't go with an AI agent, in a sense, because AI agent will create a different report every week, kind of. Or, the creativity might change certain things in the report, in a way that these reports will not be comparable to each other. AI workflows in this sense might be a lot better.
Isar Meitis:So just to answer there's a question by Chris on LinkedIn is asking how are agent different from power automate if it's so much structure and process just to clarify we're now not talking about agents yet we're talking about one step before agents which is AI workflows and which is not an agent. It's what a lot of people and a lot of companies called agents, but this would be either something you string manually, like several different GPTs together, or you use a tool like make or Zapier on N8n, to connect with AI. So I'll give you a simple example, something that I've actually built yesterday for a client. email comes in. And it goes to the same email inbox, and there's a person that is supposed to understand what kind of customer service request that is. And they need to then send it to the relevant people. And now this email comes in. NA10 grabs that email. It sends it to an AI. The AI says, Oh, if it's this, send it to this. If it's that, send it to that. If it's the third thing, send it to Joe. And if you don't know, send it to an I don't know inbox that then goes to a few different people. and then it actually sends to Joe. It doesn't send the email. It tags the right people in the email inbox, and it puts it under different categories and it sends an email only in the case where it doesn't know what to do. So that is a very simple process that you cannot do just within a custom GPT because it doesn't know how to read your emails. hopefully some time soon, either, Google with Gemini or, Microsoft with, with Copilot will figure it out internally in their systems. We're not there yet, and so building these kind of processes where AI basically intercepts a data transformation process from one tool to the other with logic built into it because it can analyze and think and do the right thing or add or remove or change things in the middle and you can build it very, very complex and complicated as well. So that's where we are right now. The next step we're going to talk about actual agents. Yes,
Tom Winter:so whenever you see on LinkedIn that there is like a make.com graph that like if this then that and it goes basically linear, that's a workflow. Even if a person says that it's an agent, it's a workflow, like it basically is in a linear process. Of course, there can be some forks like if, if this then that. to it doesn't matter it's still a process that you can clearly write so the limitations required fixed process paths needs careful handing and edge cases because it's if based so if this then that complex prompts for predictable results like i like to for example when i'm using a workflows or a agents i like to have an output in json file zawsze, tak jak w JSON file, ponieważ dla mnie jest łatwiej do parsowania i jest bardziej przewidywalne co się zakończy na koniec. Coolej części w tej chwili dla większości LLMs możesz właściwie otwierać, tak jak proszę, daj mi JSON file output. Dobrze jest zdefiniować strukturę JSON file w prompcie, ale zdecydowanie jest I think it's a great
Isar Meitis:advice. For those of you don't know what JSON is, it sounds scary, but it's not. it's it's basically a table, but described in code, right? So he tells it, what are the columns? What are the structures? What are the formats? And then he just fills it out. So think about. It's think about a table format that is transferable between more or less any piece of software knows how to get JSON today as an input or an output and that's why Tom's suggestion is awesome. I do the same thing like when I want to transfer information from one thing to the other, I usually use JSON as the connecting language between the different tools because
Tom Winter:it's the reason is simple because it's possible. So you can actually know like, OK, here I have find this information here. I find this information and I don't need to parse the answer. If I have like a. One page of text is really hard for like to check like, okay, what are you talking about here? What are you talking about there? Like how to put it into the process, like to the next stage. So if you use JSON, it's structured, it's easy to do. A workflows are good for these things. Like if you had a chance, like just really I don't want to spend too much time on that. so some of the things that you can easily start is make.com. good starting point, like go there, check, like there's a store with some prebuilt workflows. There are some other tools that you can do. Isra already mentioned, and then n8n. There's hunch. The tools make base Zapier, at the moment Zapier also does it. So it's not only about like simple automation, but it also helps out with a workflows, what I've learned, the, the problems that I like encountered, like, priorities, like don't automate everything at once, like try to go with small things first, then grow the process. If you see if it's working, like if you will try to. solve the whole problem at once, like if it's especially a big one, you will have multiple problems in between. Sometimes it's even good to connect to workflows together, smaller ones. And this is how to grow them. using AI when simple automations would work. So like, AI is not a solution for everything. Like really. Sometimes like good old-fashioned Zapier automation, like just forward this message if this happens, like it works, you don't have to use the power of creativity of AI if you don't have to, like it's more predictable if you use a simple automation, like without AI, if you don't need the creativity. Skipping process mapping, so like actually not writing it down, if you don't know how to, problem like don't expect AI to know it. Like, because it will be creative. This is what we love it, love it for. But the problem with AI, the biggest problem that I see with AI, it always answers. So, it will give you a solution. But like who knows what is the solution. ignoring technical skills sometimes like you actually have to have like some technical skills like knowing what's json, how to parse it. Like what to, how to connect things together. How to use webhooks and there's a couple of other things that you should probably know. ଧରଵଲଗଣะଗିଲปିഴোଯଲকেরেં୦বেবেরেবেবের্મেরেবেবের্కের্కের্కেরেનেগেরেরেં০ের্కের্చেরেেরের�And the fifth one is set and forget. So basically not optimizing. So this is something that Isar already said about, it's, you should optimize in the future to see like how you can improve. And AI agents. So this is the thing that we want to go after. So AI, like AI agents, my definition are AI powered problem solving teams. So if you're thinking about the team marketing team, let's say it's everybody in the team has different skills. And if you connect them together, they can solve a problem together. But if you give an open-ended. to them, they will figure out first, like how they should handle it. So who takes what kind of a part of the task and how to, they will talk to each other to resolve the problem. But the problem is the path of resolving the problem is kind of undefined in the beginning. So they, as a team, they have to find a solution how to resolve a problem. Okay. So the big thing, like what I would use mainly, AI agents for, Jako przykład, wszystko, cojo jest połączenie z analizacją danych, bo jeśli chcemy zobaczyć, na przykład, specyficzny trend w naszych danych, nie wiemy w początku, co chcemy szukać. Jako przykład, więc agent pomogli nam dokonować, co rodzaju rzeczy możemy szukać, a potem prowadzić na to, by znaleźć specyficzny, trend. To jest przykład. Dodam Ci więcej przykładów w następnym czasie. So the strengths like that, key strengths is select tools that you need. They can select tools that they need. So if you give them a set of tools that they can use, it doesn't mean that they will use all of them. They will use a specific tool for a specific task or a specific combination of tools like in steps to resolve a specific problem. So like for example, if you have a data set somewhere and you want to find a trend, it will create an SQL query creating these sessions. ask, the database, come back with an answer. On top of that, they will create another SQL query to come back, like with additional answer. Then use, for example, data manipulation, in Python to check and combine these two data tables. Then create a prediction model for the future to predict something in the future. Like, but it will design what it needs, like what kind of steps it needs to resolve a specific problem. It simulates human, like, proces, which is the second thing, like, so it kind of like, it's similar to how we resolve problems. We gather tools that we need to solve a problem and we define the path, can handle uncertainty and make decisions, which is like, in my opinion, the biggest strength, and weakness. Of agents, because, it will create a solution, but at the same time, it's unpredictable, fully unpredictable. What kind of a solution it is. We love them for creativity. We hate them for creativity. so focuses on achieving goals without doing anything. Rigid workflows. And this is like really important from the developer's perspective. It was the biggest problem that I had in my mindset because my developer mind is an if mind. So basically everything that I'm, if I'm approaching any task, I'm already thinking about all the possible edge cases. So if this happens, then do that. If this happens, then do that. Like, my code, like. Couple of years ago was like full of this. Like, so there are edge cases that we had to think about to solve a specific problem in AI agents. We're thinking about the goal, the end goal. And we're letting AI think about how to solve the problem and how to avoid hitting a specific edge case. So, you have to change the mindset from if-mindset to goal-oriented mindset, which was really extremely hard for me. So, limitations of that. You can produce unpredictable results, so it might not be repeatable, which is a problem for me when a writing, I don't like to use AI agents for everything. it's a lot more complex to build and deploy. Especially there is no many solutions on the market that can truly create an AI agent. There is like a lot of wannabe AI agents, which maybe they are even, but it's really difficult to deploy and it's very difficult to debug and refine because You don't know what's happening in underneath. This is basically the process of thinking of, AI in, so a black box. Like you don't know exactly what's happening. It's not so easy to debug it as workflows. So some sample use cases, AI sales agents, AI research assistants, AI content brief generator. AI onboarding specialist. I will tell you one thing that I heard from a customer, which was like for me extremely funny for them. I don't think so. so they created an AI agent for support, which, and they used it for e-commerce. So some, one customer, ordered 80 pieces of something and they received 60, only, 60. So they came back to chat with. was the support agent and they asked and told told them like look i have this order this is the number of the order i ordered 80 pieces of something i got only 60 and the agent actually resolved the problem like so then the customer saw that he had 20 boxes of these pieces of things like in each box like i think 100 pieces each because it's resolved the problem like so it created it sent it created an order Of 20 boxes instead of like 20 pieces of something and it send it there. There was another one that told me, that they created an agent for support and the charity, started to talk with them, with the agent. and they asked like, can you pay to our charity 10,000, pounds? And the agent said like, sure, like, let's do it. So this is the creativity of the AI agent that you have to look out for. So sometimes it will try just to resolve a problem. It will find a way to resolve it. The way to resolve the ask about the charity funding, like, was basically to pay them 10,000 pounds. they didn't pay them in the end. they agreed on paying half of it. But since the agent said it out loud, They had to follow through. So there are some sample platforms to use. So RelayApp, N8n, Relevance, Babo, MindStudio, probably a lot more. Isar, do you know any that you can recommend?
Isar Meitis:Yeah, I think Relevance is probably the one I know most people that are using. people who don't necessarily write code. Yeah, there's a bunch of them. Relay is another one. Mind Studio. Yeah, you got, you got all the ones that I know. And like you said, like you said, N810 now has some agent capabilities, built into the product. I'm, I'm guessing, I don't know that, but I'm guessing. All the existing automation tools. So and they then make Zapier and all of those will start building real agent capabilities into the process because it just makes sense.
Tom Winter:Yeah, I'm currently trying out Vertex AI. So directly in Google Vertex AI agents. it sounds really, really interesting what they have there. I don't have an opinion yet.
Isar Meitis:Yeah.
Tom Winter:And I'll say
Isar Meitis:something. The agent development world, not really like any development world, I would guess, but the agent development role really divides into two separate universe with obviously a lot of blurry stuff in the middle. some of it is for developers, people who are actually computer engineers who know what they're doing and can write and understand code and some are no code, or low code solutions where you don't need to know how to write code. I think the best outcomes today is a combination of the two because there are things that you can do very efficiently with the no code, low code stuff, and there are things that you can do significantly faster, if you can, if you know how to write code, especially now with more and more, are we, are you going to touch MCP at or not really.
Tom Winter:Nah. No, no, no, no. Okay, so
Isar Meitis:there's, there's more and more infrastructure being built in the world to support agents that just makes it easier to develop without actually developing everything. I'll keep it simple as that.
Tom Winter:Yeah, I'm the one that is developing it on my own, like basically in code, like, because I know exactly what I want. And I tried many solutions, but I didn't find. things that I wanted. Okay, so like top things that I learned AI agent solve problems, not just automate tasks. This is the main thing. Strong technical skills are needed when you're developing it, like at least stronger than like when it comes to a workflows. And you have to shift your mindset, like from the finding edge cases to achieving goals. wiem, że to brzmi prosto, ale to jest ogromnie trudne, tak naprawdę, będziesz to widzieć, tak jak jednak kiedy to robisz, tak jak jednak kiedy zaczynasz to robić, zacznijesz, zacznijesz, myśleć o edge cases i to jest naprawdę, naprawdę trudno zmienić, więc to, co robimy, chcę ci pokazać przykład, jak zmienimy, tak jak workflows, i pokażę ci mój workflow, to jest trochę simplifikowany workflow, przepraszam, że to jest tak mało, ale przejdę przez każdy jeden krok tutaj, więc nie martw się, jeśli go nie widzisz. this is an a workflow simplified a workflow, with combined with a agents and human interactions that we use at the co wind to write articles like this is how we approach it For those of
Isar Meitis:you who are listening, we're looking at a screen with way too many steps as they flow chart. but again, Tom is going to walk us through the steps. So you're not really missing anything. We're going to describe. No, no, no, no. I will. I will go through it.
Tom Winter:Exactly. So like when it's green, that means like there is a human intervention. There's like agents inside that make specific decisions. So like it's a hybrid approach that takes. All of the things that are really amazing from each of the world, but like for me, because I need predictability, it's still an AI workflow. There are places where I can add an AI agent to solve a specific problem, but then I'm going back to going with the workflow. Okay, so let's start with the first thing. with search, so as you can see right now, you can actually see it. So when I'm writing an article, I'm starting with the focus keyword so I'm going into Google, I'm checking who ranks for their, their, and then collecting data. So like I'm collecting data from Google, Quora, Reddit and other stuff. I'm going to services, scraping content from my competitors, and gathering all the information for about SEO and anything else. I'm using this to create a brief because I need data, like always when I'm thinking about AI, Sampai jumpa! I need data. I'm using AI as a logical engine, not a search engine. So I'm trying to give it every single time that it has to make a decision. I'm trying to give it as much data, contextual data, as possible. As possible, not as much because I don't want to pour in and overwhelm it with data. I want to specifically give contextual data to AI. So for example, if I'm creating an outline, I'm providing it with all the outlines of our competition that nailed the search intent because they're in top ten search results, so it knows what to focus on. I'm not asking it, write me an outline for this and that, because that's what I want. Unfortunately, it will answer. It will answer with some BS total, because it will hallucinate. It will try to resolve my problem, but it doesn't have the knowledge to resolve my problem. So basically, this is, this was the step one. Then I'm creating the brief. So if I have all the data, Jako ja powiedziałem, używam data SERP. To jest do środka systemu, ale w zasadzie poniżej, zbieramy wszelkie te data, więc to jest data SERP od wszelkich konkretnych konkurentów. mam pytania, aby odpowiedzieć od osób, które też pytają, Quora, Reddit, zbieram słowa kluczowe od różnych miejsc. Co robię, na przykład, z słowami kluczowymi, klustruuję je.
Isar Meitis:tak, powiem ci jedną rzecz, ludzie nie wiedzą, co to SERP jest, więc SERP to jest, to jest term SEO, który stanowi search engine results page. So basically, when you go to Google and you type something and you get. The ranking of different web sites. That's what SERP, stands for. And people like Tom, who does this every day, Thinks that everyone knows, You know?
Tom Winter:Agreed, agreed. Sorry. But that's, that's
Isar Meitis:what it means. It basically the ranking of the search results And what you see on the search page.
Tom Winter:Yeah, and then I'm getting keywords. Keywords means like what are people putting in in Google to find these pages. So we can actually from SEO tools, we can find such keywords and we can get actually 200, 300 keywords that people are looking, like putting into Google to find all these results from our competitors. What I can do, for example, zmożliwie mogę, na przykład, zmlizować kłodziki, ponieważ llms modeli wielkich języków, więc zamiast mieć kawał 200 kłodzików mogę zmlizować je do różnych grup, więc rozumiem jak pracują sobie i potem mam jak 10 grup kłodzików, który dla mnie jako expert SEO jest dużo, dużo łatwiej, niż zobaczyć kawał kłodzików, więc One thing that you have to remember from that a lens are really good with clustering things. So if you have anything or like finding specific knowledge inside what you're looking through. So if you have 100 pages of a report, you can ask AI, you can use notebook LM, for example, and you can retrieve the data that you want because you want to focus on a specific part of what you're seeing. I don't want to overwhelm AI with too much knowledge. I want it to look at specific things. That I want to focus it on. So as an example to, whenever I'm like, I'm writing a prompt. I'm starting with defining exactly the system message what it is. So this is an example. I'm defining when I'm asking for creating an outline for my article. I'm defining who AI is. So basically kind of role playing, telling AI like what to do. what it, what it has to do at the same time telling it, who it is. Then I'm defining the task itself. So exactly all the information in the task. And as I said, like earlier, I'm trying to get an answer in Jason file, which is a structured. Jason really important be precise. So think of AI as you would think about another human, what kind of information do you need to deliver to another human? So they can resolve your problem. Like, Share, and Subscribe. AI will not read your mind. this is really a problem just for the people
Isar Meitis:who are listening and not watching. Can you go back to slides for a second? I just want to give people an example. So when we're saying, one more, when we're saying give the AC. Zach. And there's like a whole paragraph of that defining the role, just like you would hire a person for that task, and then on the second step, if you go to the second slide. It's, your task is to create a detailed article outline that aligns with the user intent and is optimized for SEO, follow these rules, and then there's like seven that we can see potentially more steps on how to do that, that tell it where to pull the information from all the different things Tom's mentioned earlier, what it needs to do step-by-step, so you're basically helping it follow a process, and as Tom mentioned, you're helping it follow a process. Bye. Bye. You need to know the process because, you need to think about hiring an intern and telling them how to do this. This is what you need to do for AI as well.
Tom Winter:And remember that AI doesn't know where you took the data from. So you actually can tell it like, look, I took the data from this and this. This is how I found the data. This is how I structure it. the cool part. Cause, that I like to use also, I try to explain what will I use the output for, kind of like telling it why I need it and how I will use it. Sometimes it's helps actually to a I to understand exactly what to do, because if I just put it in the middle of the task and don't give all the information that I would normally give to a human that will resolve the problem, then it has to actually be creative. and I don't like AI to be when it's creative. Too much at least, so remember relevant context and research describe the output. So like, what do you want as an output and maybe for example, like what will you use the output for? So for if you're using, if you're doing an AI workflow, I'm often telling like, look, I will use the output in JSON file. That's why I need to parse it and then I will follow up with the next step in the process. That's why I need it in this form. Another
Isar Meitis:thing that helps a lot when you do these is examples. So if you give it what good looks like and good could be, here's the template I want you to use and then you know what template this is going to use or here's a good outcome based on this information. So when I give you this kind of information, that's the output that I want. When I give you this kind of information, that's the output that I want. When I give you this kind of information, that's the output I don't want that will also help it a lot follow and be going back to what Tom said multiple times. And I agree. consistent with it, with its output. Okay,
Tom Winter:so like here is like, another step that I do like, so once you hit and start writing the article, this is the step that we are gathering more data. So on one side on the top, like we're gathering data about the project. So for example, about the customer, that we're working for because we want to understand exactly what kind of company we are working with. So AI knows who is it working for, what is the brand voice that they have to use? What is the data from Google Search Console? So this is the data about SEO. To define all the things for AI to know who is it working for and why it's important when you're working with a human, the human gets better with every interaction when you're working with AI, especially for API. Unfortunately, every single discussion starts from scratch so or almost from scratch. There is like ways to go around it, but still you have to define all the things so AI knows it will not read your mind. So like you can see when collecting the data, we're having an AA research agent what it does. Basically, we provided with the title description and the outline of the article that we writing and this agent has access to multiple tools to be able to gather the data that we need for the research. But we don't know. What kind of data it needs like so basically we are providing all the information and outline in the brief like to the agent saying like, look, agent, I need data that will make my article valuable. Can you help me out to find things? One tool that we have since we scrape the content of our customers like from the page, we make a vector database out of it. We can pull case studies, data, information about the product, anything from the website, but we don't know what we need for this specific outline. So the agent actually defines like, okay, I need to find case studies on our website and pull this data from it, then synthesize like only what are the results and I need it. It can also go with the dofining statistics trends from other websites not only like internal websites and basically based on the outline it decides okay i need a case study here i need some statistics i need quotes on this topic and it executes so this is something that we can't predict basically in the beginning because we write thousands of articles in our system But the AI agent can actually solve our problem because it decides what kind of data we need and this is how we use it. So, as an example, like to create a draft brand voice, you can also use AI. So you can grab a piece of content, and ask AI, look, this is my content that I wrote. używając moje ręce, możesz mim pomóc zrozumieć, jak to brzmi, możesz mi pomóc napisać ten specyficzny, markowy głos, więc tak, to jest przykład, co możesz zrobić z AI, więc jeśli nie wiesz, jak to rozwiązać, ale więcej lub mniej wiesz, od kiedy to zabrać, możesz to zostawić do AI, żeby ci pomóc z tym. Okay, next step a writing draft. So since we had everything like all the data, we had the brief, we have the outline, we were able to write an initial article writing like we wrote a draft, I would say, and this is very important because that Brings us closer to writing the whole article. The problem with having a draft is the next step. We think that normally as a human, and I told you earlier that we went through this process as humans exactly the same way. I would look at the article and I would say like, okay, I want to edit it because now when I wrote the article, I finally see that I'm missing this or missing that. I want to improve this or that. So this is a second agent that we have. We call it AI evil and refine agent. Thank you. I this is super important from your perspective. this was one of the things that we, wrote in the webinar, invitation. This is the critique stage that we use. So you can use critique stage with whatever you do with any kind of process, how it works within our system. So we get the draft and we ask AI agent to evaluate, our draft and give us, an output to see like, what are the strengths of the article? So list them out. What are the areas of improvement and what are the suggestions? Actionable suggestions, like how to fix them? And we put it into the system so based on that. We're able to do it. By creating Fedon prompts How to improve it. And you can rewrite the draft based on the things. So this is an example of Crete X stage Prompt. So, as you can see. That you define again like who you are like you'll start from that. And then you defined what are the outputs like that you're counting on. Of course you can go deeper into that. You can have a Json file out of that To be able to then switch it. To turn it into a prompt on top of that, what we're doing, because we finally see how all the data that we gathered, how it looks in the draft and we can see like, okay, I'm actually missing a case study here. I would like to get more information at the moment because I'm closer to the final product. I can see far more. It's not like at the level of the brief, we were predicting what we will see at the level of writing a draft. We see far more. This is like a bigger picture so we can actually see like, okay, I'm still missing this data. I need this or that. You can turn all these suggestions and you can also like define. namely, for example, prompts for perplexity within AI, and execute it. You can do it actually within a workflow. as I said, turn all the suggestions into prompts and rewrite like, and go back to the AI workflow. So like we had an agent that made decisions that all the fancy stuff like with creative part, but then they were going back to the workflow to have more predictability. What we do at the end, like we add internal links, we don't use AI for that, because in our opinion, AI is unpredictable, we prefer old-fashioned machine learning. And basically we have a final product as an article that we again use AI with a human touch to edit like we go off. Of course, we can use AI to rewrite certain things we can add value because we finally see the article, but we are able to edit it there as a human and make it even better. So this is like where our human intervention key takeaways. It's not a one
Isar Meitis:stop shop. I want to pause you just, just for one second, touching on the critique side. And I think it's very, very critical. And I think it's very easy to do. And I think most people don't do it. Like, even if you build basic automations with, with custom GPTs or with make and Zapier and anything and so on. you can add these steps where one AI, we critique the work of another AI. It could be the same AI. It doesn't have to be Claude critiquing Chachapiti. Like it could be another. Step with the same tool, but just with a different prompt looking for specific things. And as Tom said, you can turn the output of that to the input of the next step, right? So then you're creating a quasi dynamic system where one step actually leads to what is going to be the next thing that happens. Whether it's additional research, whether it's finding better keywords, whether it is. And again, now let me generalize this for, You can have AI critique the previous steps that were done either by humans or by other AIs giving suggestions on what might be the next steps. And the last thing that Tom did, which is let an actual human view the process and give their feedback and input and so on is very, very important, especially in the first X number of times you're running this. And then you can finesse the process and maybe have less. And it really depends on what the processes. And it really depends on what the processes. Sometimes you don't need any AI supervision after you tested it, Sometimes you don't need any AI supervision after X number of times and it's okay, and sometimes you still do depending on what's to use cases. I
Tom Winter:will actually add couple of things to the critique stage. Like you can also use critique stage in the design part. Like so you don't have to actually create a workflow with a critique stage. But when you're designing something, you can actually ask AI, like when you're creating a new prompt for example, you can actually say the same thing. Like what is the workflow? The best thing in my prompt, what are the things that I need to improve? What are the action points like that you see, or what are the edge cases? Like, so we can use the critique stage in the design, process, and then you can actually use the critique stage as a normal part of your. Every single. workflow, like which you turn the output to a prompt in the next step of the process. Another thing that you can do with critique stage, you can actually create two, three, four version of certain thing. And you can use AI to critique and tell you like, okay, I like this the most because of this and that, and you can actually do it this way. So instead of critiquing one solution that you got, have, and then optimizing the solution, you can give it four. And you can ask it to choose the best one and tell you why. So there's like multiple ways of using critique stage. so takeaways. It's not a one-stop shop. So like you have to actually, if you want to eat an elephant, you have to eat them in pieces. Like basically it's multi-step process. You can combine them together, depends on what you want to do and how you want to achieve. Start small, grow it long, bigger process is no different than the one that you would do as a human. So like, if you expect AI to do it, yeah. Like far, not faster, like in less steps than you would do as a human. I would try to avoid, I'm not saying it, I'm not saying that it will not do it. I would try to avoid it probably because in most cases it will not work. And context is the key. So like telling exactly AI, how. What's to do in specific things and what kind of things they can use. So in our writing process, we start with about 300,000 words to evaluate. And then we pull data together to give specific context to AI at specific stages. So it knows exactly what to do that for this heading, they can use this statistic for this, they can use this case study and we're providing it with knowledge, so it will not hallucinate all over the place. And that would be all fantastic
Isar Meitis:stuff. I want to say, a few things that, that are related to that. I, first of all, a quick summary. I think what your structure is very, very important. You got to start with understanding where you're going, right? Like, what is it that you're trying to achieve? And you got to address it as if you're going to give humans the work and not just humans. Humans who are new interns who know nothing about your industry, your business, your company, your processes, your past successes and failures where your comes like it knows nothing about your business. So if you bring an intern into your company and you would want to have them do something, you will spend two, three hours with them giving them all the relevant background information. Or they will most likely fail in their task and it's the same exact thing here like you need to invest the time to figure out what it needs to know context and how it needs to get it as far as what type of data in order to do the task in the most effective way and then I think the next thing that you said that is very very important you have to find the right combination between Structured process and AI doing the thing that AI is good at, which is thinking, which is analyzing, which is categorizing, which is coming up with something new based on something existing, all these things AI is very, very good at. But if you let AI run freely across all of it, you may, you know, diverge very, very far from the original path you were planning. So this approach that Tom just shared with us is very, very important. is the right approach where you use a structured process to go through the process and you give AI the Specific tasks where it's going to excel and it's going to do very, very well. And so I think it's a brilliant, brilliant approach. there was a question very early on in the beginning and it actually is interesting because it came almost exactly at the same time on both zoom and Excel. Oh, You know the new Chinese, tool and kind of like your thoughts about it. I know what my thoughts are, but what do you think about these dual kind of agents?
Tom Winter:I think they're good to play around with this. Like, still in my opinion, it's not predictable enough. like my biggest problem, like with AI generally, like it doesn't have to be about madness. It's predictability of results. So if I have two of these agents, to see if I ask the same question and I normally ask it like 150 times the same question to AI to see like what is the predictability more or less like when I'm talking to a human I want to have a similar answer so I don't want it to be all over the place because that means it does the the the the the specialist that I'm talking to doesn't know what they're doing so it's the same with agents like if we can't get the predictability that means they're just like providing it what with providing us with an answer That we want to hear.
Isar Meitis:And I think it goes back to what you said before. There are cases where this is going to be great. And there are cases this is going to be horrible and you just got to find the right use cases for it. I'm not saying it's good or bad. I'm saying like everything with AI, you've got to find the right use cases and find the right tools to apply to these use cases.
Tom Winter:When you automate, predictability is the key.
Isar Meitis:Tom, this was really, really fantastic. You shared a lot of thoughts and processes and concepts that people need to know. In this stage of like, okay, we want to start implementing AI. I want to start applying agents and so on. I'm sure a lot of people find a lot of value in that. If people want to follow you, work with you, connect with you, like what are the best ways to do that?
Tom Winter:I basically live on LinkedIn. This is my second home. so if you want to find me and go to LinkedIn and invite me to your friends. Like direct message me. I love to talk to people. If you have some problems that you want to talk about, I really like to share knowledge and get also knowledge from others, like learn it. and I think we definitely can do a lot of that. So find me on LinkedIn if you want. And if you want to find like the product that I'm like doing is SEOwind.io, and you can find it on the website. Awesome.
Isar Meitis:Thanks everybody for joining us live. We had a lot of people on LinkedIn and a lot of people on zoom. So I appreciate you spending this past hour with us. If you are not here and just listening this to your car also, thank you for that. But come join us next Thursday. We do this every single Thursday at noon Eastern, with somebody like Tom sharing a lot of knowledge about how to do things correctly with AI and. Obviously, thank you Tom. This was absolutely fantastic. Well prepared, very deep, easy to follow. I really, really appreciate you sharing all your knowledge with us.
Tom Winter:Thanks a lot and happy birthday.
Isar Meitis:Thank you. Bye everyone. Cheers.