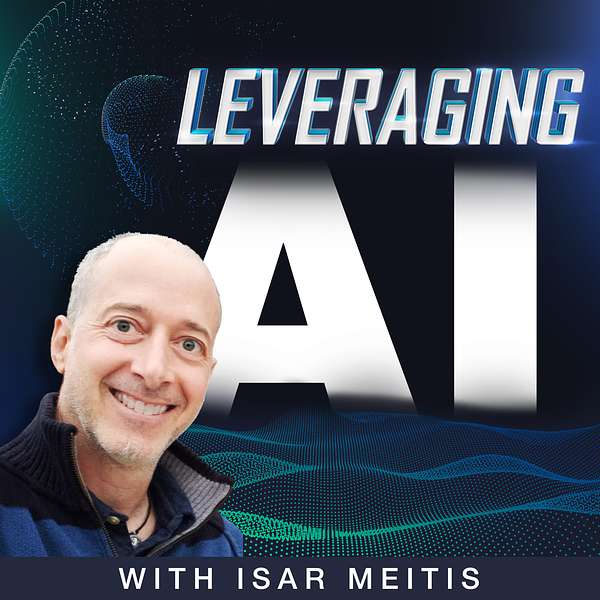
Leveraging AI
Dive into the world of artificial intelligence with 'Leveraging AI,' a podcast tailored for forward-thinking business professionals. Each episode brings insightful discussions on how AI can ethically transform business practices, offering practical solutions to day-to-day business challenges.
Join our host Isar Meitis (4 time CEO), and expert guests as they turn AI's complexities into actionable insights, and explore its ethical implications in the business world. Whether you are an AI novice or a seasoned professional, 'Leveraging AI' equips you with the knowledge and tools to harness AI's power responsibly and effectively. Tune in weekly for inspiring conversations and real-world applications. Subscribe now and unlock the potential of AI in your business.
Leveraging AI
173 | ChatGPT vs. Google Colab in the ultimate data analysis showdown with Isar Meitis
Data-driven decisions = better business results. But what if you don’t have a data science team or technical skills? That’s where AI comes in.
In this hands-on webinar, we’ll show you two powerful ways to analyze business data—using ChatGPT and Google Colab—without writing a single line of code, or having any technical background. Whether it’s financial data, customer insights, geographic segmentation, etc. you’ll learn how to turn raw data into actionable business intelligence.
We’ll walk through step-by-step, real-world examples to help you:
✅ Unlock deep insights from data—fast and without coding
✅ Compare AI-powered analysis using ChatGPT vs. Google Colab
✅ Make smarter business decisions that drive real results
No tech skills? No problem. Just bring your curiosity and get ready to transform the way you work with data!
About Leveraging AI
- The Ultimate AI Course for Business People: https://multiplai.ai/ai-course/
- YouTube Full Episodes: https://www.youtube.com/@Multiplai_AI/
- Connect with Isar Meitis: https://www.linkedin.com/in/isarmeitis/
- Join our Live Sessions, AI Hangouts and newsletter: https://services.multiplai.ai/events
If you’ve enjoyed or benefited from some of the insights of this episode, leave us a five-star review on your favorite podcast platform, and let us know what you learned, found helpful, or liked most about this show!
Hello, and welcome to another live episode of the Leveraging AI Podcast, a podcast that shares practical, ethical way to leverage AI to improve efficiency, grow your business, and advance your career. This is Isar Metis, your host, and we have. A topic today that is really dear to my heart. I've always been through my entire career as a senior executive, a data freak. I always wanted to know what's happening in my businesses and how can I make better data-driven decisions in my businesses. The business company that I run was last minute travel. I had an amazing team in last minute travel, and when we started building our technology platform, the first thing we did. Is to build a data management system that allows us to capture data from multiple siloed sources that we had in the business before and bring them all together so we can understand exactly what's happening across the entire business. Back then, that was very unique and almost nobody had something like this, but. It helped us really grow the business from 20 million to a hundred million in a few years just because we could understand exactly what's happening in every aspect of our business and just make better decisions on daily and hourly basis on conversion and traffic and costs and execution of different aspects, and creation of code and deployment of code, literally every aspect. Of our business was monitored and we could see exactly what's happening and we could make better decisions. So my personal experience as well as now as a consultant with different companies. Having the right data is half the battle, but then knowing how to analyze the data to actually get insights that can support the business is the different half of the data. And that's gonna be our topic of today's episode. We're going to dive into how to use the data that we have in the business right now in order to make better business decisions and to get the insights that we need in order to make these decisions. Now. I will say that many companies have. Data that they're not using and you're like, they're not using it or they're using it in a limited way for several different reasons. Reason number one is some people are just lazy and say, okay, we have these reports and we have these dashboards in the tools, and it gives us enough information, and we're good with that. So that's reasonable. One reason number two is that. People just don't know what insights they can get from the data, and so they're limited with what, where they can go with that. Reason number three could be, that the data is siloed in different places, so they have some data in the sales department, some data in marketing, some data in hr, some data in finance, et cetera, et cetera, et cetera. And they have no means of combining it together. Reason number four, why? Why people are not making the most out of the data is they know the insights. They have the data, but they just don't have the in-house skills. Sometimes those in-house skills are as basic as we have somebody who has an Excel whiz who knows how to put stuff together, but in many cases that's not even enough. And some pieces, some businesses don't even have that, but. In some cases, that's not enough because to go beyond, like when I was running last minute travel, I had a data scientist and even a senior and a junior data scientist working and analyzing data and running through the information that we had, on top of that infrastructure that we built. And so if you don't have that, you're still limited with what you can do. But AI actually comes to solve all of these things. So how do we solve these kind of problems? Now, before we dive into that, if you're in here with us live either on Zoom or on LinkedIn. Please come and share who you are, what you're doing, where you're from and so on, so you can get more benefits out of it. In addition to just listening to me talk about data and how you can make better decisions. let's get started with some data analysis and knowledge that you can gain. So in ChatGPT, there are amazing capabilities to do data analysis. Now the truth is you can do this in multiple tools, but the trick is how can you do this in the most efficient way, and how can you do this in the most valuable way possible? And what I mean by that is. Gemini knows how to analyze data. Claude knows how to analyze data. ChatGPT knows how to analyze data and some of the other tools as well. Some of them do better than others. Now, the question you need to ask yourself first and foremost, is what kind of insights you're trying to get. And then once you understand those insights, what do you want the output to be? The output could be a dashboard, the output could be a chart, the output could be a report. The output could be a fancy report that includes charts and graphs and text and everything. So it really depends, and you gotta know all these things in order to decide exactly what you want to do. And so once you have that, you can then, You can then identify what is it that you're trying to get from the data. And one of the cool things with chat PD is that because you are running within not a traditional data analysis environment like power BI or one of those, you're actually running within a chat that can understand the. Context and understands the conversation, and understands the process, you can actually do much more interesting things than you could do otherwise if you are just using a standalone platform. So what I wanna show you as a very first step is how you can take raw data and in ChatGPT, convert it into a very detailed report on any topic that you want. Now, that particular report that I'm gonna show you right now is going to be. About how to create a analysis of a publicly traded company. But you can do the same exact thing with any data that you have. You can do it with sales data. You can do it with marketing data, you can do it with HR data, literally whatever you want. So let's look at this. What we're gonna start now is, first of all, I'm gonna show you ChatGPT and we're gonna walk through a very long and interesting process on how to go from numeric data in the table to a very detailed report. What I wanna show you in addition to the report itself is I want to show you that the. Report itself is like a 30 page report with graphs and charts. And for those of you who're listening will not be able to watch this. But for those of you who are not listening and can watch this, after we're done, I will show you the actual report. But what did I start with? So you can start with several different data sources. The right one to start with is starting with a CSV file. Why do you wanna start with a CSV file is the fact that a CSV file different than Excel is actually not as heavy as an Excel file. So what you wanna start with, if you wanna start with a CSV file while CSV and not XLSX for several different reasons, but the main reason is that. AI tools, all of them, ChatGPT included, are can read CSV files a lot easier than they can read all the other stuff when you're creating a CSV file to drop it into ChatGPT, they are best practices that you need to follow in order to make the best out of it. The very first thing that you want to do is you wanna make sure. That the formatting is as simple and plain as possible As humans, we like creating tables that have different colors that have subtitles every quarter that have a gap in as a blue line between Q1 and Q2. That is actually a column in the Excel that we color in blue. We like to add three different tables on the same page because it allows us to see different things. Altogether, we add three different headlines. Headline one is quarter that merges three cells together. Underneath that you have months and underneath that you have weeks. And so there's three different headers for everything and so on and so forth. And for us humans, it's very easy to read that and follow For the ai, it's really, really confusing. So the best practices is have one header line. If you need to include the quarter in the month, then put the first sale as Q1 dash January, then Q1 dash February, then Q1 dash March, et cetera, and so on and so forth. More merge your sales. Do not put any totals, and definitely do not put any sub totals in the middle. Of either the rows or the columns, because that confuses the AI as well. It will add that as just another number and it'll throw away your results. make sure that using consistent formatting, meaning don't use a number in one cell, in a text, in a different cell, each column should have the same formatting, across the entire column or across the entire row, so the AI knows how to read it in the most efficient way and if you are using a multiple tab Excel file, if you save it as a CSV, each and every one of those tabs needs to become its own CSV.'cause CSV has just one page. So you need to export each and every one of them separately as A CSV and then upload all of them to ChatGPT. So that's just best practices to get good consistent results. What I've done here is actually something a little different. I've started with a publicly traded company and I went to their website and their website actually has their financial analysis that looks like this. and you can see that it's just a regular standard. Financial analysis information that they have on their website, but I couldn't find a CSV file to download. I'm sure if I worked very hard, I could have done that. But the cool thing about chat PT and all the other tools as well is that you can take a screenshot, which is what I've done, and then I've uploaded to chat PT and I said, please turn the data into a table we can analyze. And then it created a table. In the same exact formatting, with the same exact data in it, and it's actually very good at doing that. So if you've never done this before, literally now, every time I need to move information from one place to the other, I take a snapshot, either a screenshot or with my phone, and I ask ChatGPT to convert it to data, either text or tables or whatever I need. And it does a fantastic job every single time. So then I have, Table of data, and I have, you know, Q1, Q2, this year, that year. total revenue, cost of revenue, gross profit, operating expense, operating income, all the financial data that exists in a standard p and l of a company and with different years and with trailing 12 months. In this particular case, like I said, this could be any data that you have in your company. And then I went ahead and. Gave it the first prompt. You are an expert financial analyst and I need your assistance in reviewing the performance of this company. Please let me know what are the key insights you can see in the data. So the way I like to start every time I bring data into Che GPT is to give it a very broad, general question. I usually ask what information you can find in the data and what general insights I can get. I do this for two reasons. Reasonable. One is it allows me to understand that ChatGPT. Understands the data. Reason number two is that it helps me in getting ideas because sometimes it will have ideas that I don't, and then I can see, and then I can see what it. Gives me a suggestion of oh my God, I didn't even think about that. That would be great to know this thing. So I always start with that. So the answer that I got is actually a pretty good start to the analysis. So it says, okay, revenue and gross profit trends revenue rolls from 526,000 in fiscal year 220 to 578,000 in fiscal year 2022 by Dipped in 2023. And gross profit and key takeaways. And then it went to operating expenses and operating income with a few bullet points on that, and then large negative in 2022. So it basically told me there was a large dip in 2022. The data shows, stark swing to a net loss in fiscal year 2022, losing$31 million compared to profit and all the other ones. and the same year 2022, the total. Unusual items were 48 million, so this is like one of the things that happened. Then it talks about recovery of net income and earning per share in 2023 and 2024, and interest and expense and leverage considerations and EBITDA fluctuations. A really great report that I didn't have to ask for any specific details. It just gave me the report, and you can do that. Like I said, with any data that you give it, it's just a general start and then. I said, okay. I'm very curious. This is me prompting. I'm very curious about the one time large negative in 2022. These results are for a publicly traded company called Superior Group Companies, SGC. Can you access their 20 22 10 K? So the beauty about doing this kind of analysis in ChatGPT is that it has access to the internet and I can ask it to go and grab additional information. So it did. It helped me identify what are the reasons, so we went and got their 10 k, their actual, reports from the SEC. He was able to identify what were the issues, so then I gave it a specific link to their 10 K and the information in it, and then it pulled information, it still had issues with understanding some of the data. So I downloaded the 10 K as a PDF, and I uploaded, and then it found the answer and it gave me a very detailed reasoning how, what's the data in there? I also ask it to provide me with citations. Where it found the data. So what I'm looking at right now, I'm gonna read to you that I was just listening. It says, summary of reason, declining fair value due to market conditions, cash flow decline, and stock price decrease. And then there's a detailed. Actual explanation that is a quote from the 10 K. So I'm not gonna read all of it to you, but I'm gonna read a short segment of it. It says, in conjunction with the Resegmentation during the second quarter of 2022, the company performed a goodwill impairment analysis and determined that the estimated fair value of previous uniforms and related products, blah, blah, blah, blah, blah. And he tells me, because I asked it to, on the next column it says it's page 28, section called Goodwill Impairment. Charge. Why am I sharing all of this with you? If you are giving it information, ask for specific quotes from the original information and ask for the reference, the page, and the section it had taken the information from that is true, not just when you're doing data analysis. That is true, even if you're writing summaries or anything else, because it will allow you to very quickly verify that the information that you got is correct. All I have to do is to go to page 28 in that section and find that segment, and I can do it with a command F because I have the exact quote from that section. So it helped me find all of that information and then I said, okay, great, thank you. I would like you to help me with creating some graphs. Let's start with a line graph of the total revenue year over year, including, the TTM. So it did that. So it did, a graph that I'm looking at right now. Again, those of, you're not listening, it's just showing revenue, over time on the X axis, including the trailing 12 months as the last number. Then I asked it, about more specific stuff and I give it more information. And then I asked for another graph that was very interesting to me. So please create a graph of the total revenue compared with the graph of the operating expenses because it felt like they're not growing in the same pace. And it did, it created the graph for me. Now. Then it was still not very, very clear. So because they're very different from one another, one line, the revenue is very high and the operating expenses are relatively low. So I asked it to create a ratio between them. Again, that's a parameter that doesn't exist. So I prompted it to please create a graph that shows the ratio of operating expenses compare with total revenue, and what we can see in this graph that from 2020 to. 2023 and then continuing in the total in that trailing 12 months. The ratio grew from zero point 26 to 0.35. A very big jump in operating expenses over those few years. That's data that is not obvious unless you can literally ask a question and get a graph in front of you. Now I'm gonna scroll down and there's more and more and more information, and I created subsets of tables and I asked it to go and get the current trading. Value of the stock and it actually, ChatGPT knows how to present it in a live graph that you can move your cursor over and actually see one day, five days, one month, because it's pulling the data in real time from the stock market. So you can see that in the report as well. And I created a lot of other graphs and bars and so on, but then gets the interesting part. How do you turn all of this into an actual helpful report? So the prompt was you are an expert data analyst with 15 years of experience in writing reports about publicly traded companies. I would like you to tell me what the outline should include in a report about the company we just reviewed, which is SGC. The target audience are senior business executives with, knowledge in this company. The reports, the report needs to cover the basics. But also provide unique insights that may not be obvious. Please take your time, review the information again, and let me know, what should be in the outline. I would like you to cover the topics that I asked about, plus additional things that it suggests, and it created a beautiful outline that includes, an executive summary, business overview, financial performance, operating expenses, profitability trends, stock performance. Deep dive in 2022. So the things that I asked about in the beginning, it has like three or four bullet points in that balance sheet and capital structure, industry market positioning, all of that on its own, like it created a great overview. What I asked it to do then is I asked it to create this in canvas. So. Canvas, it allows you to then see the response in a way that is editable by you. So if you open a canvas, and those of you who don't know canvas opens a side by side kind of environment. In chat GPT, you create it by either asking to write something and when it does it, you can literally just ask it to open it in canvas. So when you open canvas, it allows you to see it on the right and continue the chat on the left. And the benefit of that, the other benefit of it is that you can edit the document that is in Canvas and you can, and you can ask questions and add information in specific segments. So this is what I did afterwards. I literally, I. Highlighted in canvas section after section. And when you highlight it, you can ask chat GPT to relate to just a section. And then I ask for more and more details in specific topics until I had the full report. The one more thing that I added that I really love doing when I create these kind of reports is I asked chat GPT to create a placeholder for each and every one of the graphs that we created before. So ChatGPT in Canvas. And by the way, none of the other tools knows how to put. The graphs inside the text that it's generating. So it's generating a fantastic report that has multiple pages and is formatted perfectly that you can work with collaboratively. You can go and change information, you can add your own text, you can highlight specific sections and add for additional information about that section so you're slowly building a deeper and deeper, more and more detailed report. But then, you don't have the graphs, so what I'm asking you to do is I'm asking you to put placeholders for the graph. So you can see here because of the prompt, it says visual line graph showing the revenue decline across segments for 2023. And then in square brackets, which is what I ask it to do, it gives me the name of the chart that shows up in the regular chat. So on the left side where I have the chat, if I'm looking now at one of the graphs, you can see it has a name and I ask it to put the name in square brackets. As a placeholder where it needs to go. So the only thing I need to do afterwards is to actually go to a regular Word document. And if I click on that, you will see the final report this now after I export it in into PDF. But all I have to do is get the report, add a header, add a footer, and add a table of content and everything else, and I'm gonna show you now, this page, this is like 14 pages long, and it has all the information that we had before, plus the graphs that I had to manually paste in the right place. But it was very easy for me to do because all I have to do, it tells me what's the name of the graph. I have to look for it in the original chat, copy, paste it into the report, save it as a PDF. A work that used to take a team of researchers a few days to create, I can now create in probably 20 to 30 minutes. Now I can do the same thing with any other kind of report that I want. I can do this, like I said, with financial reports, with hr, peer reviews with. Marketing data from multiple systems and combine it together. We talked about some of the limitations that we have as far as siloed data in companies. This solves that. So one of the use cases that I did with one of my clients that we're trying to analyze the actual cost of specific clients versus the revenue from specific clients, so the revenue lives in one system. Most of the cost actually comes from. Customer service, which leaves in another system, and there was no way for them to combine it together. And literally all we had to do is export the data from both systems, drop two CSV files into chat, pt, and ask questions. And then you get a comparison of the revenue versus the amount of customer service you do per client, which tells you. The real overhead of that client compared to how much revenue they're generating, which allows you, again, going back to insightful information, to segment your customer service to different tiers like platinum, gold, and silver, and then give them different level of support based on their. Revenue that they're driving to the business or the profitability they're driving to the business, which is not easy to figure out unless you have either a data scientist or a tool like this that is basically a data scientist in a box. And the reason I'm saying that, if you go back to CHE pt, the way CHE PT is doing all these things. Is it's actually creating the code to run each and every one of these prompts. So when I'm creating a prompt in ChatGPT and asking it to create a table or a segment or anything, what it's actually doing is it's actually writing code to make it happen, which allows me to, if you know how to write code, which allows you to go and see what's the code and how it's doing it. But in addition, it allows it to be accurate, which is. More important because instead of Chet and all these tools are not great at math, by the way, they're not great at math because they are statistical models. They get it. 95% correct, which in doing math is not a good idea. But what they're actually doing here is they're writing Python code in order to get the results. So this is ChatGPT, but let's say we wanted to go a step further. Let's say in addition to just doing data analysis, I wanted to do something more interesting. So I was looking to another use case and that use case actually came to my mind because. Google came out with a new tool or actually with a release of an existing tool with updates, and that tool is called CoLab. And what is Google CoLab? Google CoLab is a way for people with data analyst experience to create very detailed reports without infrastructure. So it's basically data analyst infrastructure in the cloud that you don't need to know how to install, you don't need to run it locally and so on. You can go ahead, drop your data in, and it knows how to do everything in the backend as far as the infrastructure, as far as setting up databases and calls and APIs and all these kind of things. This was a very, this was a great tool for data analysts, but not necessarily for people like me because I don't write a single line of code. I don't know how to do that. But what they added last week is the ability to prompt the tool to write its own code, which is really cool. So let's, so what I wanted to try is, I want to try a use case I couldn't do in Chachi piti. One of the things that I thought of is, let's say I wanna start a business that serves gyms in my area, and it provides them. Whatever. Either support to fix their gear or the actual equipment itself or anything else that Jim may need. Now, I wanted to make it interesting, so I tried it first in chat, pt. I said, what if I go to chat PT and I try to create this thing? So let's look at cha GPT To do that, I need to know what are the gyms in my area, right? So let's say my business is in this zip code 3 2 8 0 2, which is somewhere in Orlando. And let's say that I want to. Know what gyms are in the area. So I said, okay, create a table with names, addresses, and latlong of all the gyms within 20 miles radius from the center of this zip code, and it created this. But it's really bad. And it's really bad because it gives me like seven gyms out of a lot more that are out there. And what it did, this is actually, I did it with several different models. I also did it with the reasoning models. And what it's basically telling me in the reasoning models says, I don't have real time data access to this, so I'm gonna try to do my best. But I'm telling you in advance, it's gonna be a limited kind of information. okay, that's not great. So that's definitely not all the gyms in that area. So how can I solve this? So now let's look at CoLab. CoLab looks like a very geeky environment, but don't be alarmed when we look at it because I will show you exactly how it works. And the way it works is all I had to do is I had to open CoLab and I had to go and it's like everything in Google. it's colab.research.google.com, and then you go to file and you create a new, notebook or whatever they call it. New notebook and I created a new notebook, and then all I had to do is prompt it. The prompt that I wrote is create a table with names, addresses. Lat. Longs. Exactly the same thing I wrote to chat pt. But what it did is it wrote code to connect to Google Maps API, and it asked me to get an API key, which was a little scary in the beginning'cause I didn't know how to do that. But after about five minutes on YouTube, I got an API Key and I, what it did is then it queried Google Maps. To get this data and then instead of getting the five that I got before I got 59 gyms, and then you've got all the information for all these gyms. It put it in a very ugly user interface, which is not very helpful to me. So I wrote in a prompt, I said, can you create this as a interactive table? So then he wrote code to put it in a table that actually makes sense and look like humans would wanna look at. So now I have different columns. I have an index, which is basically gym number 1, 2, 3, 4. It has the name, it has the address, it has the latitude and longitude. And the reason I wanted those is for the final step, which we'll get to in a minute. So the same kind of exercise could be for any brick and mortar business. You want to know how many schools are in your area. You wanna know how many people live in your area. You wanna know whatever you wanna know as far as geographical data. This knows how to get, because it knows how to write a code to go and connect to third party APIs and get the data. Then I said, okay, this is cool, but let's say that I don't wanna work with large chains like LA Fitness and Planet Fitness because they already have that solution in house. They don't need me. So what I ask you to do, and I literally just clicked a button and said, can you embed this as a Google Sheets in the same work environment? It did, and the cool thing that it did is two things. First of all, literally I now have Google Sheets in collab, so I don't have to go to Google Sheets, but it also gives me a link that I can open this as a regular Google Sheets and work in a regular Google Sheets, but it feeds, I. Into my notebook, but again, the fact that it knows how to do it here is really, really cool. So now I have this in Google Sheets. Now I don't know how to write code, but I know how to use Google Sheets. So what I did in Google Sheets is I literally wrote a simple formula that will tell me if the name of the gym is Planet Fitness or LA Fitness, and it will say yes or no, depending on a simple search, like something that every person hopefully knows how to do in Google Sheets. Now I ask it to take the data from Google Sheets. And again, I did this by prompting it. I said, get the data from the interactive sheet, and then it updated its own database. So now you can see that it in its own database. That is again, this ugly format. It actually added this additional column that I added in Google Sheets. This whole process took me about 10 minutes, including figure out the API. Now where the real magic happens. So now I have a table that is active in Google Sheets that is integrated into a data scientist environment that is working with prompts. And my next prompt was using this data, create a Google Maps to display all of these gyms, highlighting the ones that are not large chains in green. And it wrote a piece of code and it created the map that is 20 mile radius from where my. Made up office or warehouse is with green and blue dots on the map that shows me the gyms that I want to serve, or maybe where I would want to open my next location based on this research or so on and so forth. My next step, which I'm not gonna show you, but I'm gonna talk about, well, what you can do, I can now ask it because it has API to Google Maps to create the best routes to cover. 15 gyms per day in the most optimal way. So I don't waste gas and time and money and it will give me a different route every single day to cover different gyms every single day of the week. So in five days, I can cover all these gyms in the most effective way, and it will write code and it will do that for me, and it will give me the navigation for each and every one of these gyms. This is nothing short of magic in my eyes because it allows you to do stuff that regular data scientists will take a while to do. And you can do this without being a data scientist. Now, yes, you need to be a little bit geeky, and yes, sometimes it writes the code and it fails. And I will show you examples, but what happens when it fails to do the code? And you can see an example here. It tells you, okay, do you want, an explanation of what is the problem? And you say Yes. And then it opens another sidebar and it explains what the problem, and said, do you want me to fix the problem? yes. And then it creates a new piece of code that you can then deploy into the other side not knowing anything about what the code does, because I don't know anything. And then keep on going until you make it work. Summary of what we discussed today that I find to be absolutely incredible. one is data is what drives businesses. if you know what's happening in your business across more and more aspects of the business, and you can go deep, you can make better decisions, making better decisions will drive better results for the business. The hard part is knowing how to analyze the data. These two and then actually doing the actual analysis. What I showed you today is two different options on how to take different kinds of data. Some of it is internal data, some of it external data, like the locations data, and bring them in into data analysis tools that are either free or almost free that you can use. At scale to do stuff that previously was impossible to do unless you had a data scientist in-house. And even then they probably have limited bandwidth and they can't do everything. And in the same amount of time, that will take you to write an email to your data scientist to explain what you need. You can actually do the analysis itself in ChatGPT. Just get the CSV file. Follow the best practices that I shared initially and drop it into chat, and then just ask questions. It will generate analysis, subsets of the table, new columns to the table that do really sophisticated math to get to them. Create bars, charts, whatever you need, and then you can even ask it to write a report for you that you can just copy and paste into a tool. Or if you want to go a step deeper, use a tool like collab that you can prompt to do stuff that brings external data. Plus you can obviously drop your own data. You can still take a CSV file and I've done that as well. You can take a CSV file, drop it into collab and said, analyze this and you will write its own code and do things and give you answers. What I found in that particular use case that ChatGPT does a better job. So you get. Better outcome because it understands the context. It's not just asking it to do a specific type of code. It understands what you're trying to do in chat pt, which collab doesn't. So you can do very geeky things in collab. But as far as analyzing just a CSV file chat PT will get you better results faster and with less hassle. So if that's your use case, go to chat GPT and use it over there. That's it for today. I hope this was helpful. I can tell you. For myself, I use this all the time with whether my own data or when I'm working with different clients. I will have to say one thing before we go. You have to know that if you're not using a paid account of ChatGPT and you're asking them not to train on your data, then they will train on your data. Meaning if your data is data secrets or client sensitive information or anything like that, and you do not know for sure that the model that you are using will not use this straight up for training, do not upload the data to that platform. Now there's some data that there's no problem uploading to the platform because. It's not really sensitive, and you can still do that. Another way to do this, the right way to do this is to obviously get a license level that does not train on your data. You can do this even with the regular$20 a month cha, GPT. There's a form you can fill up on the Cha g PT website on the OpenAI website and you can Google it and you can find it, to ask them not to train your data. And then you get a written confirmation in an email saying that they're not going to train on your data. That's number one. Number two is, let's say you have data that, and you don't have access to a tool like this and you don't wanna risk the data. You can always declassify the data. So take your entire data sheet and divide it by 27.6 and then it's not the real numbers. And then do a final replace to the customer's names to client one, client two, client three, client four. Do all the analysis you want, run the reports. Do everything because then it's data that is not really the real data. And when you bring it back after you write the report and you're now in Google Docs or in Microsoft Word. Do a finery replace again and change all the numbers back and all the names back to the original numbers. So that's another workaround. But all I'm saying is be careful and aware with what you do with sensitive data because you might be exposing it to whichever platform you're uploading it to. That's it for today. Really hope you found this helpful. Have an awesome rest of your week.