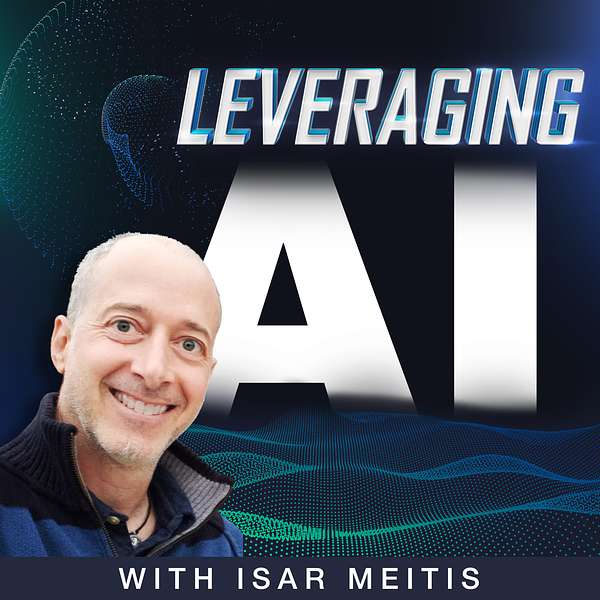
Leveraging AI
Dive into the world of artificial intelligence with 'Leveraging AI,' a podcast tailored for forward-thinking business professionals. Each episode brings insightful discussions on how AI can ethically transform business practices, offering practical solutions to day-to-day business challenges.
Join our host Isar Meitis (4 time CEO), and expert guests as they turn AI's complexities into actionable insights, and explore its ethical implications in the business world. Whether you are an AI novice or a seasoned professional, 'Leveraging AI' equips you with the knowledge and tools to harness AI's power responsibly and effectively. Tune in weekly for inspiring conversations and real-world applications. Subscribe now and unlock the potential of AI in your business.
Leveraging AI
122 | Dashboards on Demand: Mastering AI Tools for Rapid Business Insights with Isar Meitis
In today’s fast-paced business environment, spending hours sifting through data and creating reports is a luxury no one can afford.
This live webinar is your fast track to mastering the art of turning raw data into powerful, decision-driving dashboards—using the cutting-edge AI tools.
I will walk you through, step by step, how to harness your existing data from CRM, ERP, and marketing systems to create visually compelling dashboards that deliver actionable insights. Learn how to generate weekly, bi-weekly, or monthly reports in mere seconds—so you can focus on what truly matters: making strategic decisions that drive your business forward.
Join us and discover how AI can elevate your reporting game, helping you and your leadership team stay ahead of the curve.
About Leveraging AI
- The Ultimate AI Course for Business People: https://multiplai.ai/ai-course/
- YouTube Full Episodes: https://www.youtube.com/@Multiplai_AI/
- Connect with Isar Meitis: https://www.linkedin.com/in/isarmeitis/
- Free AI Consultation: https://multiplai.ai/book-a-call/
- Join our Live Sessions, AI Hangouts and newsletter: https://services.multiplai.ai/events
If you’ve enjoyed or benefited from some of the insights of this episode, leave us a five-star review on your favorite podcast platform, and let us know what you learned, found helpful, or liked most about this show!
Hello, and welcome to another live episode of the Leveraging AI podcast. This is Isar Meitis, your host, and we have an exciting show for you today. We're going to talk about one of my favorite topics, which is data analysis and making data based decisions, which is important in my eyes is one of the most critical aspects of being successful in a business. So a lot of people take or make business decisions based on gut or their knowledge or their understanding. When the reality is most businesses has lots and lots of data that can help them make educated, data driven decisions that by definition will achieve better results. This is true across every aspect of the business, whether it's sales, marketing, HR, finance. And you name it, every aspect of the business operations, manufacturing, if you have data of what's actually happening and you know how to analyze it, you can make better decisions and hence, improve the product success of whatever process that you're trying to do. The problem with data analysis is that we quote unquote are somewhat lazy. And the reason we're somewhat lazy is because every platform we use out there gives us some kind of data analysis. So if it's your CRM, if it's your marketing automation, email platform, Inventory management, financial platform, whatever platform you're using, it has X number of reports that comes with it. And we just got too used to living with these reports. In better cases, you have somebody in your company who's really good with Excel, and then some of the stuff happens. Either manually or quasi automatically, and you can take the data from that tool and then run it into an Excel thing that you already have ready and get additional benefits and additional capabilities. The problem is there are many cases where we want to know more. We want to get specific insights about specific things. We're going to look at different examples that are available in many businesses. We're going to look at them today. And we don't do that because it's just a lot of work and we have a lot of other stuff to worry about and to take care of. And what I'm going to show you today is that by using different AI tools, you can get both tables as well as charts and graphs of basically anything you want to know if you have the data. And we all have access to that data. So being able to use AI tools to do data analysis in a very accurate way and save yourself hours of report generation. And that happens in every single company, salespeople, finance people, and so on, spend. An hour to half a day a week every week in every single business in generating different types of reports, either for themselves or for their higher ups so they can show what happened in different channels. And all of this can now be automated or semi automated with AI tools while getting better data analysis. So we win on all fronts. What I'm going to do, I'm going to share my screen. And we are going to get started with the actual how to do the things that I just talked about. Those of you joining just on the podcast, I'm going to explain everything we're seeing on my screen. So if you're driving or walking your dog and you can't watch the screen right now, that's perfectly fine. You can listen, and I'll explain everything that we're doing on the screen, but feel free to join us. We do this every Thursday live on zoom as well as on LinkedIn live. I'm going to actually start with an example that we're going to dive to in the end. And the reason we're going to dive to it in the end, because it's the most advanced stuff, but I want to show you what's possible. So what I have right now is I have open in front of me, A page of Claude. And what Claude has is it has a tool called artifacts, which allows you to see or write code and see the outcome of that code right there and then on Claude. But there's also a tool in Claude that's called projects. And what project is these mini automations that you can create that will allow you to run them again and again. So what I'm showing you right now is I have a Claude screen open in front of me. Those of you who are listening or watching and don't know what Claude is. Many people know ChatGPT. It's just a competitor to ChatGPT. It's a large language model. It's powerful. really good at several different things. One of them is what I'm going to show you right now. So what I have is I'm going to drag in here a file that is an export of one client from a CRM. So it's going to have the, of a specific account. Everything that's under that one sales person's accounts, different clients that he's managing, pipeline items and so on. So it's basically a pipeline report out of the CRM for one specific account manager across multiple clients that he's managing. So I drag the file in there and all I do is I click go. I don't type anything. I don't prompt anything. I just drag a CSV file in there and I click go. And what you're going to see on the screen Is that it's going to start writing code, it's actually going to write a code that's going to generate a dashboard that is replacing the hand made, report that this, Sales manager had to write before and the dashboard will have all the different things that he needs in order to present this to his team, to his boss, to himself, whatever he needs to do without having to do anything other than dragging and dropping CSV file into Claude once a week and click go, we'll give it a few more seconds to run. Cool thing about this, and you already have, if you're watching this now, you can see the dashboard on the screen. So we have total pipeline value of 33 million. Average deal size, 2. 802 million total opportunities, 12, and then it's divided by the different types of the business, which domain it comes from in this place, it's not defined. I have a pie chart that's showing the opportunity probability distribution. So zero to 20%, 21 to 40%, 41 to 60, 61 to 80, and 81 to a hundred percent. So basically how many. deals we have in the different probabilities of success. And then another graph that shows me how much money is in each probability for success. And all of this is happening. And on the bottom, there's an even more interesting thing on the bottom. There's a decision support table that suggests what I would do with each and every one of the accounts. So the first account, for accounts that are really close to closing, I should focus to close, consider moving to the next quarter, consider moving to two additional quarters out, and the way he does this, I will show you afterwards in the end, because as I said, this is a little more detailed and sophisticated than some of the things we're going to look into, but I wanted to show you how incredibly powerful this could be right as we get started. All I had to do to see this dashboard that has a lot of detailed information, both in data as well as charts, as well as decision making support matrix, by dragging a CSV file and clicking go, and about 30 seconds later. I have a functioning dashboard that I can also publish and give access to other people. So let's go back to the presentation and I'll really start from the beginning. If you are on this call and you find this interesting, I would love your feedback on would you like to know how to do this. If you're listening to the podcast, just wait till the end and we'll get to that as well. there's already a question. How does Claude know what to do with the file? so we're going to get to that at the end of this episode. I promise. As I said, this is a little more And guys, I hope you can follow along with me. And please, love, care, and support. And I'll see you in the next video. Two types of data analysis that we do. We do qualitative data analysis and we do quantitative data analysis. They're both very important, but in this particular case, we're talking about data, like numeric data, quantitative data analysis, and in the company, I want you to think for a minute, how much quantitative data you have in your business. And the reality is you have. So if you want to write in the chat, if you are on LinkedIn or on zoom, just write in the chat, what quantitative data analysis you have in your business, but I will start sharing here. And really, if you're driving and listening to this, I want you to think about it for a second, but there's multiple aspects of the business that has quantitative data. And that varies from financial information, obviously, sales funnels, how many people are in different stages of leads and different stages of sales, and how many proposals we've sent, how many we won, how many we lost, customer service performance, how many tickets were open, how many tickets were answered, how many were closed, and how much How many calls did we get? Inventory, how much inventory you have of each type? How long has it been sitting on the shelf? How much value is in their marketing? How many ads have we sent out? Conversions, funnels, et cetera. Compensation plans of employees, employee performance and evaluations and so on. It's really endless. Every business have to do this. An endless number of data points that are all quantitative data. Now, as I mentioned, for every business, You have the data. The data lives in some kind of a system. It lives in your CRM. It lives in JIRA. It lives on Monday. It lives in, financial platforms, HR platforms. it lives in all these places. Almost each and every one of these platforms Gives you some reports, but not all of them, but all these platforms allow you to export the data as CSV, right? So you can take the data that you have and export it in a format that you can open in Excel. And that's what most people do today. If you want more than what the platform will give you, you will open it in Excel. In many cases, you even know what you're looking for, right? You know what additional information, what additional insights you want to find from that data. Sometimes that's not even true. You just want to know, I have all this data in my sales system or my CRM or my inventory or ERP or whatever. I want to learn more from it. What else can I learn? So sometimes we don't even know, and so even if you do know, don't always know how to look at that information, right? So what actions do I need to take to find the additional information I want to get? But if you dive a little deeper, then there's questions you want to ask, let's say in this particular case about CRM. What actions do I need to take to close these existing opportunities that I have? What is the total value of pipeline that I have right now based on the probability that I have? These are just CRM questions. What should we focus on to maximize this specific opportunity we have right now? So we have 12 or 20. Which one should we focus on in the next two weeks versus which one should wait for next quarter? all these things are sales related, but the same questions can be asked about every aspect of the business, whether it's manufacturing, whether it's inventory management, HR, and so on. So let's look at a few examples on how AI can help us take raw data and analyze it beyond What we can find in the built in tools. So the first example that I want to give you is a CRM example. And what we're going to look at is pipeline data and how we can analyze it. So I'm going to open in this particular case ChatGPT and we're going to scroll all the way to the top and we're going to take a look at what we have. So what I've done here is I took an, A CSV file that I exported out of my CRM and I wrote the following prompt. You're an expert data scientist with 20 years of experience in pipeline and sales data analysis. Please review the attached file and let me know what data is includes, what data it includes and what insights can we gain from it. So you can see I'm starting with a very general question, meaning I am not telling it specific things that I want to find out. I am not giving it specific information. I am just telling it to tell me what it finds. And I do this for two different reasons. One, it gives me new ideas that I didn't think of on information or insights I might find. But two, it helps me understand that it Understands the data. So think about it before you would give this task to an intern in your company or even your head of business intelligence. If you have a new type of question, you want to understand that they understand the data that we're looking at. And so I always start these processes by asking a general question. And what you can see is that it analyzes the data. Tell me everything that's in this data set. So idea, account name, opportunity, name, type, opportunity, domain, field. Of, the field it's in, probability, close date, open date, opportunity size, like all the information that exists in this data file. And then it tells me insights that we can find, opportunity scope, opportunity types and domains, sales pipeline health. And for each and every one of them, it gives me a description of what that means. if you don't know what sales pipeline health means, it means the mix of probabilities and forecast categories can provide a health check of the sales pipeline. High probability opportunities in later quarters might need more attention to ensure closure, and so on and so forth. It gives me more additional ideas on what kind of insights I can gain. And the whole point is going from data to health. to knowledge, to insights, right? Because insights are actionable. Data is just the raw information that doesn't really tell me much. So let's continue. So what I've done then, I wanted to know what information can I, what decisions can I make based on the information that I have. So based on where We are in the quarter. Are we in the first week of the quarter? Are we in the middle of the quarter? Are we in the last week of the quarter? And based on the probability of the opportunity, I need to make specific decisions. And in the business, each business has its own guidelines or rules of thumb of what to do in different steps, but you can build a system that will do this. So what I've created, I created another file here that just exists as a Word document. And that Word document shows the matrix of what to do. So if you're in month one or the quarter, What to do in month two of the quarter, in month three of the quarter, what to do, and for each and every one of the months, the data shows what happens if the probability is less than 25 percent, 25 to 50 percent, 50 to 80 percent, and 80 to 100 percent. And you can see it's basically a table, a matrix that shows what to do in each and every one of these scenarios. And underneath that, there's an explanation with an example. So the example says, if we are in the second month of a quarter, and the probability to close an opportunity is 40%, the salesperson should move the close date of the opportunity to the next quarter. So it literally just looks at the cell to explain how the table works. So I attach that as another reference to ChatGPT, just uploading another file. And then I gave it the following prompt. Let's start by focusing on account one because we have multiple accounts. This is obviously fake data just for the purpose off this, presentation in your life. It will be an actual name of an account. We are in the month of July of 2024. Our quarter ends at the end of September. I would like you to use the attached and then I take the name of the document pipeline status decision making matrix and create a table with the following columns. Mhm. Suggested action, opportunity name, probability, current close date, suggested close date. So all the information I want to see either for myself to make the right decisions or that I want to present to my boss or as the head of the department that I want to share with my team so they can execute based on that. And then I explain what each column is. So suggested action, is based on the attached pipeline status decision making matrix. Opportunity is the opportunity naming data set and so on. I keep on explaining each and every one of the columns so the AI knows what it is. And then I run it. And it created a table that shows for just the first account, so account one, so one account manager, each and every one of the opportunities, its probability, its current close date, its suggested close date. So the suggested close date is if I. If the suggestion is to move it two quarters out, what is the new close date, two quarters out or one quarter out and so on, or if it's not changed at all. And then it gave me the list of each and every one of them. What you can see in this table is that it's all over the place because it literally went by whatever order the data was versus based on what makes sense as far as decision making. And so I ask you to change it. I'm like, please aggregate based on the first column. In other words, merge all the cells in the first column that are the same action and keep all the other columns as separated rows. and then it created as fixed table. So in the fixed table, what I have is I have all the opportunities that I need to push to close, which are between 80 and 100%. And I have four of those in my table. And then I have five opportunities under the category of push hard or move to next quarter. So you can analyze them one by one and you know which ones you can have a higher chances of closing. And then I have consider moving to next quarter. I only have two opportunities. So this is a really powerful tool to help you with decision making based on a rule of thumb that your company has. That you can, don't have to do manually to present again, either to your team, to yourself or to your boss. You can literally do this in seconds, time and time again, once you have this up and running. I then went further ahead. I said, great work. Now let's do a broader pipeline analysis. Let's go and look at all accounts. I would like you to show me a chart that shows how much money we have in the pipeline for the current quarter categorized by probability. And I told it which probabilities I want to have. So less than 25%, 25 to 50, 50 to 80, and 80 to 100. That's just the way we look at opportunities for our business. And you can see that it created a chart that shows me how much dollar value I have in each and every one of the probabilities. That shows me that I have a huge amount of money, too much money in 25 to 50%. So relatively low probability, I have I don't know, about 30 percent of the potential pipeline of the business. And all I had to do is ask the question. So I uploaded a CR, CSV file and I asked questions in plain English and it generates the chart for me. Now, yes, you need to know how to tell it what you want in the chart, but literally explain it like you would explain to an intern or a data scientist that you have. what do you want on the x axis, what do you want on the y axis, what do you want the ratios to be between things, and so on. And it will create the chart, and if it's not exactly what you wanted, you can continue explaining in plain English what you want. So let's continue. So now I know I have too much money. in lower probability. So what can I do next? So I said, okay, great. Now I would like you to show me the 25 to 50 percent category, just that category where I have a lot of the money and show me the opportunity size broken down by account name. Use a similar bar chart as before. So it created the bar chart for me, So there's a question by Rosemary, if you can do similar things within Google Sheets. Yes, you can do the same within Google Sheets, and thank you, Rosemary, for asking. The way I do this in Google Sheets, and I probably need to do a complete separate session on that, because it will take us a full hour to go through that. But what I do in Google Sheets is I integrate AI tools through their APIs. Into Google sheets. I actually do it through a third party platform called Open Router and Open Router has access to multiple large language models and each and every one of them has pros and cons and what it's good at and what it's not. And I can pick it. Four different stages of the analysis, different tools to call from within a cell. I know that sounds really a magical to some people and many really confusing to others. But yes, the short answer is you can do this, a similar thing in Google Sheets while bringing in. Large language models into Google Sheets itself, and you can even use it for qualitative data analysis to analyze customer reviews, to analyze emails, to analyze proposals, and so on. And I'll maybe do a separate session about this, in a few weeks. That's not a bad idea. So thank you, Rosemary, for asking that question. so now let's go back to our thing we asked. Chachapiti to, and by the way, to do this kind of analysis, Chachapiti does it best as of right now. And the reason for that is Chachapiti has a data analysis capability. And what it does in the background is it writes Python code. So every time I ask it to do something, which in this case is to Take the category of 25 to 50 percent opportunity and give me the accounts, the specific opportunities in each and every one of them. It will write Python code in the background, will execute it, and then will share the outcome with me, which guarantees that it's going to be an accurate outcome. So what we see here. This is, as I mentioned, it's fake data. In the fake data, I created one account with a lot of data that I can play with in my different presentations. it ruins my graph because this one has 130 million in pipeline as far as account 1 and all the other accounts are really small on the bottom and I can't really see what's going on. all I did is literally ask it a simple question. Please remove account 1 from the graph. So that removes account one and that now scales all the other accounts to something that we can see. So what we see in the graph right now is we see that between 25 and 50%, we have most of the money broken into two potential client accounts. In this case, it's account two and account 30. So now I'm like, okay, now I know where I need to focus my sales team's efforts because these two accounts, only two accounts, that's it, holds the most of the money that's stuck in relatively low probability. So the next obvious thing is to understand what opportunities are in these two accounts that we need to try and push forward. So it's literally what I asked for, and now I'm asking for something a lot more granular. Please create a table showing all the data from the original data set, but only for account 30 and account 2 that we have identified based on a graph. And What you can see is that it did it. So now I have a granular level broken down table that shows me each and every one of the opportunities that I need to look at in order to work either myself or with my team or to show my higher ups that we need to focus on in order to increase The probability of our pipeline and the whole process took me about five minutes, knowing nothing about my CRM, knowing nothing about how to do it in Excel by asking simple questions in English. This is can be applied to any aspect of the business. As I mentioned, you can bring in a CSV file for your employee reviews and the scoring that they've done, that they've given to each and every one, given to each other. You can bring your financial data and do the same thing. You can bring your final information and do the same thing. Just ask questions in English. You're gonna, you can build tables, you can build graphs, and all that information is available to you. And then you can export that information as a CSV if you want to do additional analysis. or just copy and paste information to wherever you want or just use it as is. So this was just one example. I want to show you another example from a different field in the business, just to get an idea what else you can do with this. So if you're, this is going to be relevant to you. If you're a software company. But if you're not a software company, it doesn't matter. I'm going to explain how this is relevant. So in this case, I took information from Jira. Jira is a platform that most software companies are using in order to manage their development. So what features are being developed, what bugs are being opened, what bugs are closed, and so on. And in this particular case, I gave it the following prompt. You are an expert data analyst. in the SAS industry, look at the attached data and let me know what information you can find in it and what business technical insights can I gain by looking at this data? Same exact concept as before. I want to see that it understands. So it gave me all the data that's in there, a very long list, and then it gave me ideas. on what kind of information and insights can I gain? Priority and severity, status, aging, suggested actions, improve external quality control, and so on and so forth. So it gave me a lot of ideas. So then I ask it the question, which I really doing every now and then. So we know bar charts and we know line graphs. And we know pie charts and that's where it ends for most of us. But the reality is there's dozens of types of different graphic representations that you can use to show different things that you may not think of because we don't use them regularly. But these platforms like ChatGPT, they know all of them. So I asked it, what would be the best graphics way to show the time it took to close different defects by severity. So it gave me ideas. box plot, histogram, line graph, stacked bar chart, or scatter plot. Each and every one of them, it explained what are the pros and cons for each one. So I can choose which one is right for me. I can pick all of them and see which ones makes more sense. But then I ask it to create it and it created the code. And the first two ones that it gave me actually did not make sense to me. So then I ask it for something else. I said, please create a stacked bar chart with dates as the x axis and the number of defects created by each date. The stacked bars should show the priority with medium as yellow, high as orange, and urgent as red. And it created the bar chart for me. And what you can see again in my fake data, That we have more and more defects as we move forward in time, which is not a good thing. You can also see the severity of them and so on in very clearly just by asking in very simple English. But here we have it by day. So it's still, there's many bars and it's hard to see the details. So what I did next. As I ask it, please aggregate the X axis data by week. And I have the same thing by week, which gives us a much clearer view. Again, if you are with us live, either on LinkedIn, and I see there's a lot of people on LinkedIn, or on Zoom, and you have any questions about any of these steps, that's your benefit of being with us live, that you can ask questions. please do. But let's continue. So now we see a bar chart that showing us that it's the number of defects we have are growing week to week in the last month and a half or so, and they grow dramatically in the last two weeks, which will allow us to dive in and see what's happening in these past two weeks. And I could have asked for any other analysis. You can aggregate by month. You can aggregate by topic. You can do each and every one of those things. But what I want to show you is I want to show you how to make it even more sophisticated. So let's go to a different example, still in JIRA, still in tracking defects of our software. This could be, again, applied to anything else in your business. What I'm doing in the second example is I'm going to combine different sets of data into one analysis to get a better understanding that's not available From one platform. So I started with a similar thing. You are an expert data scientist working at a SAS company. I need your assistance in analyzing software defects data using the attached data. As a first step, please create a new table. So I'm taking the old data that I have, and I'm creating a simplified table. All the all that I need out of the 20 columns in the original data is defect. I. D. Priority E. T. A. In days date created. and date closed. And so it created that table for me. And then what I asked it is I asked it to calculate. Now, please add another column that will calculate the number of days between date created and date closed. So if you think about what I have right now, I have the original data that has a projection from my development team telling me when they think the DFIT will be closed as far as ETA. But I also have the date it was opened and the date it was closed, so I can calculate how much time it actually, and this comes from two different data sources. But since I uploaded both files, I can do that analysis. So now I have, in addition to the ETA column, I have a new column that I didn't have before that's called time to close. in days as well. And what I can do now is I gave it the following prompt. Now I would like you to create a bar chart with the y axis being the different priority categories and the x axis being the average days. For each priority category show two bars. One is the ETA, so basically what my developers told me it's going to take. And the other, the time to close, meaning how much it actually took. And what you can see is that it's created a graph, but it created it flip flopped, meaning what I asked for the x axis is the y axis, and vice versa. And you will do it every now and then. So all I had to do, Afterwards is ask it to please flip the X and Y axis. And he did. And now I have the graph that I wanted that is showing me something that I don't have access to because it comes from two different platforms. I can see how much time my developers thought it's going to take to develop the solution for whatever defects, and I can see the actual time it took them. And I can see that there's a huge discrepancy, mostly on medium level bugs. And I can see that there's a huge discrepancy, mostly on medium level bugs. And now I can go and drill deep, drill deeper like we've done previously in the previous example and say, okay, show me all of those. And I can try to deep dive and see why there's such a big discrepancy. I can do the same thing for any type of analysis that I wanna do. In this particular case, we have where the data is coming from. Is it coming from us, meaning our QA team, or is it coming from our clients reporting these bugs to us? And I can keep dissecting the data more and more just by asking questions in plain English before doing this, before having my. AI consulting and education agency. I was running a large travel company. I was running a very large operation, a hundred million dollars in sales, multiple people across multiple departments. And I had a business intelligence team with two people, a senior and a junior person that would put all the data in the databases and write queries for us and create dashboards. Like any business intelligence team does to get this kind of information would take me two or three days. And the reason it would take me two or three days is because I would I would have this idea. I want to see the difference between how long. We thought it's going to take to how much it actually took. So I would send an email like this to my BI guy. And he would say, okay, let me get back to you tomorrow. And he would write his Python code and he would write a script to get the data. And he would come to me the next day with the first graph. And then I would either have not, it's not exactly what I wanted, or I wanted to ask a follow up question and that will take another day. Now, I have no BI team and it takes me literally seconds to get this kind of information by just dragging and dropping files into different AI tools and asking them the right questions in order to get any level of details you want. Now I'll say something else specifically about ChatGPT and its data analysis capability. One of the limitations that we have using, a data set that is really big, the tools. Like Excel or Google Sheets have a limited number of rows they can manage. I don't know the exact number, but let's call it 15, 000. But let's say your data set has a million rows. You just cannot upload it to Excel. Excel will crash or won't even open or will tell you it can't handle it. And if you upload 15, 000 rows, which is its limit, it's going to run very slow. Those of you who have tried it know exactly what I'm talking about. I've uploaded data sets to ChatGPT to do this kind of work of more than a million rows. And it works and you can dissect the data and ask any question and I've uploaded multiple data sets of hundreds of thousands of rows and was able to create insights by combining them together. So it's an extremely powerful tool that is impossible to do unless you have a BI team and somebody who knows how to write script or you pay 20 bucks a month and use chat GPT. So let's continue to additional. examples and move to the more sophisticated one. But a quick summary. So far, most of the tools you have the ability to export CSV files. All you have to do is drag the CSV file into ChatGPT, tell it that it's a data expert with whatever deep knowledge in the field that you are in and ask it simple questions in English. Tell it what insights you want to get or ask it. what insights you might find, and it will be able to give you that information as well to give you ideas on what else you can look for. And you can combine multiple data points, multiple data sources, and just get charts, graphs, tables, anything you want in order to make better educated decisions. Okay, let's continue from here. So remember that example that I showed you in the beginning, I want to show you how it's done. So if you remember what I've done, or if you joined a slate, all I did is I dragged a CSV file into Claude, hit go, and didn't give it any instructions, and got the outcome. So how is that done? And the outcome was a very detailed dashboard. That I can use for multiple things. So I've done this in several different steps, and I'm going to show you all the steps so you can do it yourself. So the first thing that I did is I opened Claude and again, I'm using the paid version of Claude. If you're not using the paid version of these tools, please do like I'm paying for literally all of them. I'm paying for the Gemini pro. I'm paying for Claude. I'm paying for perplexity. I'm paying for chat GPT. And the reason is each and every one of them is better than the others in something specific. And in this. particular case, it's artifacts by Claude that is nothing short of magic because it runs the code and executes the code right there on the screen. So you can go back and forth and back and forth and correct it without knowing how to code. And I don't know how to code. So those of you like, Oh, I don't know how to code. I've never written a line of code in my life. I don't know how to read it. I don't know how to debug it, but I do know how to use it within AI in order to create magic. And so let's see what I've done here. So get the paid version so you can have artifacts so you can do these kind of things. It's worth every cent. So here's the prompt that I used. It's a slightly longer prompt because it's a three step process. But you're an expert data developer and data scientist working with a sales department of large corporations. Your goal is to develop a web app that does three steps. Data upload, and it gives me, I give it some instructions. Data analysis, and it gives that some instructions. And then dashboard creation. You're a data scientist with 20 years of experience working with sales teams. I would like you to use the pipeline data from the CSV that is now in the Database and turn it into a highly interactive dashboard, including many features, filters, sliders and tabs as necessary to enable a sales director and also regional managers and VPs, VPs of sales to get as many insights as possible. Also use the pipeline status decision matrix, so the same file that I showed you yesterday, I've attached here as well to assist in making decisions for the current pipeline. And you can see here on the right, the files that have attached to it and the information that I gave it as far as the CSV files that I gave it and then it wrote me what can be done. So it created a plan sales pipeline analysis web app. Step one data upload user interface. You define what that could be, what the back end could be. Step two data analysis. database design, data processing, step three, dashboard creation, user interface. So it literally broke down what I give it into a lot more details on what needs to be done in order to create this solution. But then I'm like, okay, let's get started small. Let me help you with uploading sample data. Please review the attached data, refine your approach to the database as a dashboard definition accordingly. I'm helping it and understand what kind of data I'm going to provide to it in order for it to better define the requirements. And then refine the requirements and gave me something more specific to the information that I gave it. So now it's a lot more granular and a lot better tailored. Not by me giving it a long explanation, just by giving it the data set. So it understands on its own, what is that data? What are the insights that can be gained from that data? And created a updated description of what can be done in order to learn from that information. Thank you. So then I requested it to actually create the file to create the dashboard. So great work. I would like you to make a few changes. Oh, so let's start with this. So I asked you to create in that case. Let's try to create the dashboard, assuming that the CSV I gave you is the CSV that the user uploaded. So I'm basically running a test case after it gave all the definitions and I went back and forth. I'm saving you the time, but there was a little bit of back and forth in defining, exactly what I want in the dashboard. And then it created a sales pipeline dashboard. And I have a drop down menu of the different divisions. So I ask it for filters. So now I have division 1, division 2, division 3, division 4, division 5. So this one, it's not for all divisions, but I can use a filter and filter just for one division. You can see the dashboard I didn't ask for it. It created this on its own based on the logic that it thought that would make sense. I can ask for additional dashboards, by the way, for those of you who know how to read code, if you click on the code button, on the preview thing here, it will show you the code. So then you can go and read the code and see what it is. But what we have in the dashboard is total pipeline value, average deal size, total number of opportunities, pipeline division. And then opportunity probability distribution. And then all I did is I went back and forth and added more and more features to the dashboard. And every now and then it just didn't work. It failed. And maybe if I click some of them, so I click now one of them that shows you. And instead of showing me a better dashboard, it says. Unsupported libraries or icons detected. Basically, there's a problem with the code. But I didn't write the code. I don't know how to write code. What do you do? if this was the first response you would have gotten, you probably would have said, this thing doesn't work. I don't know what the hell this guy is talking about. I need to stop here. all I'm doing in these kind of cases is I'm copying and pasting the comment that I got when it fails to run the code back into Claude and asking him to fix it. And sometimes it wills and sometimes it won't, and sometimes it will get a different problem. And then you keep on copying that until you solve the problem. And this one probably took me, I don't know, an hour to go back and forth. And you can see it's a very long conversation. so those of you who can see, those who are just listening will have to believe me. But it's a long conversation. But in the end. I had a very detailed dashboard with all the components and all the capabilities, including the recommendation matrix, all built into this dashboard. So that was step one. Now to use this or reuse this, there's two ways I can do this. One is I can now figure out the prompt of all prompts that will include all the components that I've done from all the little prompts along this entire conversation and save it as a prompt library item and then use it again and again. But there's an easier way to do that. And the easier way is to use projects. So what are projects? Projects are mini automations within Cloud, just like those of you who know GPTs from ChatGPT. So Cloud has projects, which is an automation that you can build within Cloud. So how did I build the automation? The first thing that I did is I took the code off that final dashboard. So again, those of you who are not watching this but listening to podcasts, on the left, in Cloud Artifacts, I've got the conversation. Me. Writing something, Claude writing back, but on the right side of the screen, I have what it's actually doing and in there's like a toggle button. I can see the actual dashboard or I can click to see the code that it has created on its own in the background. So what I've done is I copied and pasted this code into a word document. Or in my case, a Google doc, but it doesn't matter an external place. And again, I know nothing about how this code works, but it works. It generates the dashboard every single time. And so what I did is I copied the code into a document and then I created a project. Now the project is actually really easy. It's another set of instructions that it knows how to run. So on the right side here, you can see that I have two different things. I have my instructions. So my instructions, which are basically a prompt for the project, are relatively short because most of the code was already created when I created step one. So let's look at the instructions for the project. You're a data scientist with 20 years of experience working with sales teams. Your goal is to receive a pipeline data set from the user as a CSV file and turn it into a highly interactive dashboard. Once the user. Provides you with a CSV file. Carefully follow the following steps. Copy the attached code. So I attached that document that I told you. Of the code that I didn't write. That I worked back and forth with Claude to write. copy the entire code. Replace the example data from the attached code with the entire data set from the CSV upload by the user. And then I even told it exactly the syntax of the example data in my example file, right? So I literally copied and pasted a section of the code that shows it how to grab the data and told it to replace it. I then said, with the actual opportunities from the users uploaded CSV, you must upload all rows from the user data. Do not write a comment suggesting that importing all rows, actually import all of them. Why did I write that? I wrote that because when I ran it the first time, it brought in four rows out of 150 and said, and wrote a comment in the code saying, keep on importing the rest of the data. I'm like, no, I actually want the whole data. And so we will do weird things like that. And then the same thing I did with asking it to continue running the entire code because every now and then it would just run. Part of the code. So I had to give it these examples, but the way I've learned, I tried several times. I tried once, I tried twice, I tried three times. and every time it had an issue, I added something to my instructions. So whether yours needs to be exactly like mine or a little different, the idea here is iterations, repetition, fail, try, fail, try, fail, try. You're like, okay, this is way too much work. I could have done some of this in Excel and be done with it. Yes. Maybe it would have taken you less time to do it in Excel once, but I, as I showed you in the beginning of this session, all I have to do right now, every time I want to update is to export the data and hit go, and then I have to do nothing, I can read another email, and about a minute later, I have the dashboard ready to go based on my needs, I'm the one that tailored what graphs, what charts, what tables, what recommendations, what's going to be in it for the usage, and then Of me and my team, and so that's basically how I use this for myself and for my different clients. You can see that attached is the code so you can see the attachment and now I can run this as many times as I want in a few seconds. So now a quick summary of all of it. We have data. We can export it as CSV. You can use multiple AI tools. I just showed you two specific examples. One of them is within ChachiPT. The other is with Cloud Artifacts and Cloud Projects. But in all of these cases, I can reuse what I'm learning the first time. And yes, the first time you're going to spend more time and you're going to bang yourself, bang your head against the wall, say, I don't know, this is not really working. And I'm getting all these errors. Invest the time, because if this is something you invest an hour a week to do as far as data analysis and writing reports, invest five hours in creating this automation, because then you can do it once five hours, and then in week six. It's pure ROI of not ever having to ever do this tedious work again, either you or your team and so on. I see a bunch of questions coming in. I'm going to answer them. So Troy is asking, does Claude have a permission to reuse the data you uploaded to further train its capabilities to provide to other clients? the answer is no, but you can do this on your own, meaning I can go back to my first step in this process and take, okay, here's new data. I also want to see this. Let's try to combine this into, into the data set. Let's start combining this into the dashboard or whatever the outcome is that you want to be. And build that and then all you have to do in the projects section, meaning the automation section, just replace the code. So once I have the new code, after I tested it a few times, I can export that, replace the reference file within the automation, and that's it. The automation will keep on running just with the new capabilities. there's a question from Danielle. This might be a silly question. No such thing as silly questions on this show. how do you get it to work? How do you get it out of the code and into a format to send to relevant team members or do the team members just view the specific model? Oh, great question. so there's several different ways to do this. option number one is to give everybody in your team access to this, tool within Cloud. And then they drag the file and they run it. And so they run it within Cloud and there's no, no problem. Option number two, you get any open source. code execution platform for this type of code. And that's just depends which language you're using, but there's multiples of them and give those to your employees, or you host them on your intranet so people from within your company can run it. And then they don't have to open cloud. They just open that tool and they just drag their file in there. And the thing is going to work. Option number three is to use some kind of a wrapper. So there's multiple tools that will allow you to build apps with no code. And literally, if you think about this app, all you need is a place to drag the file to. So there's, it doesn't need any fancy user interface, but it's just going to say something like, drag your CSV file in here. And there's going to be an arrow and people can drag the files and it's going to execute the code because in the background, you created an application and there's multiple tools today that will build these applications for you literally in minutes without writing any code. great questions. Another question from Jennifer. Do you give your clients access to the dashboard or you're just providing them reports? it depends. It's totally up to you, right? If you want to build this as a client facing tool, yeah, you can build this as a client facing tool and then put it behind whatever username and password for just your clients to see instead of the rest of the world. or don't, and just give them a screenshot of that on weekly basis. You can do it. Either way, it will work. A question from Sandra. What do you think the strong points are of each gen AI platform? Wow. Okay. that's another hour, but, I really use the different tools for different things. And that keeps on changing because they keep coming up with new models all the time. So about. Every month or so, I would go back to some of my use cases and test them across the other platforms. One of the tools that I really like using, is a tool that's called, Chat Hub. And Chat Hub is a tool that has, multiple of these LLMs running in parallel on the same page. Screen and it's a Chrome extension. So it's not anything you need to install. It just runs on Chrome. So it's a Chrome extension that you can grab and run up to six. I think on the paid version, I always use four. That's what I got used to. So you can run Lama three, Chachi PT four, Oh, Gemini 1. 5 pro and Claude 3. 5 sonnet and give it the same prompts. And see the results on all of them in parallel. And it's just like magic. And I do this for two different reasons. One, it allows me to really test how the latest models are doing compared to one another on very specific use cases. And two, when I just need to ideate, I want to get an idea for my next episode. I want to get an idea for my next post. I want to have an idea on how to address a specific situation with a specific client based on EOS. So I will go and open chat hub and I would say, okay, we are using EOS as a company and I want you to help me, workflow with the current problem with this kind of client. Here's some information about the client. here's what I'm trying to achieve. And that will get four different answers. All follow the EOS methodology that all are based on the information that I gave it, but coming from cloud gemini. So from an ideation perspective, I get more ideas just doing it once because I'm running it. Practically on four, different tools. So that's how I do this. It's very hard for me to tell you right now, which does what things better, because even if I do tell you this might change next week. So what I'm telling you to fish instead of giving you a fish, if you, I hope that helps, but just keep on testing them regularly for different use cases that you have. and you'll be able to know for your particular use case, what works better. At that moment. Awesome. I really appreciate you guys joining me. Those who have joined us and ask questions on LinkedIn and those who join us on zoom. If you're not doing this, please come and join us. This is awesome. You can see it's interactive. I'm answering questions. you can see the screen and everything that I'm doing. And so I really appreciate you spending time with us and thank you so much. I will see you next Thursday. And those of you who are listening, keep on listening to the Leveraging AI podcast and share it with people who you think can benefit from that. Rate the podcast. So if you're here on LinkedIn, by the way, or on Zoom, and you're not listening to the podcast yet, go and sign up and, for the Leveraging AI podcast where you get notifications every time we come up with a new show. Not all of them are live, so there's more content on the podcast than they hear on the live shows. That's it for now. Transcribed Thank you everyone. Have an amazing rest of your day.